Digital Life Seminar Archive
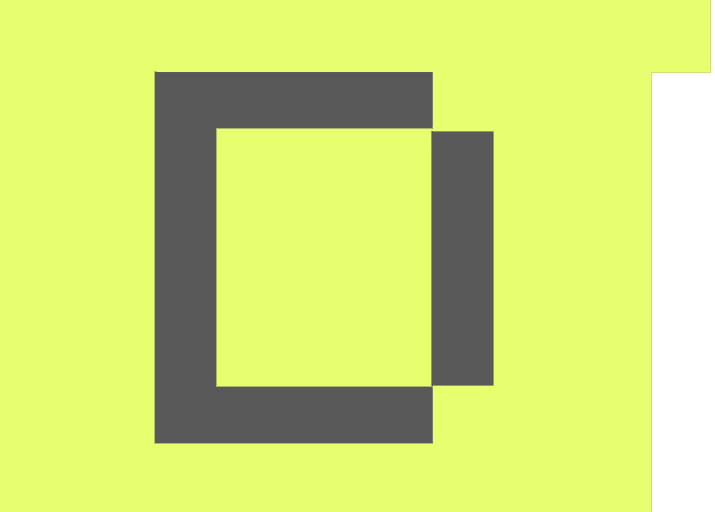
Liane Huttner
University Paris Saclay
Defining Automated Decisions in EU Digital Law
The concept of automated decision-making (ADM) is central to European digital law. Qualifying a decision as automated entails the application of some of the most discussed and important rules in the discipline. For instance, the right to explanation of a decision based on an AI system found in article 86 of the AIA applies exclusively to automated decisions. Similarly, the right to obtain human intervention in article 22 (3) of the GDPR only exists when the primary decision is fully automated.
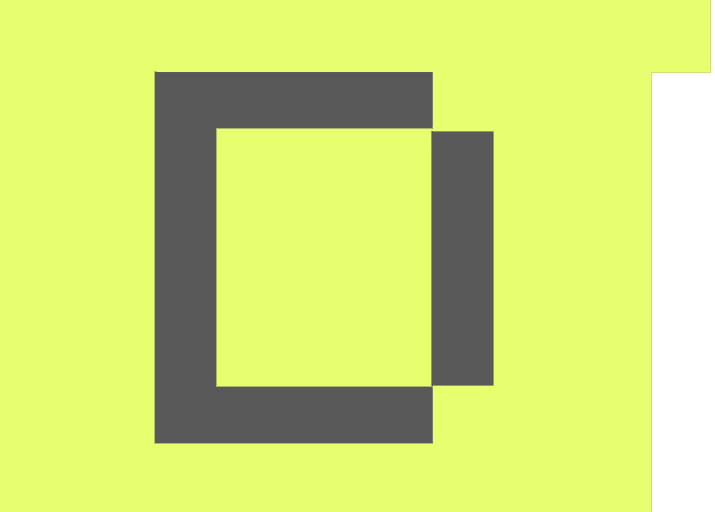
Lily Hu
Yale University
How Race Makes a Difference
A tension appears in contemporary social-scientific studies of the causal effects of race. Race is understood by most scholars today to be a deeply social phenomenon—acategory that not only explains distinctive patterns of social inequality but is defined by these myriad social differences. But this fact about race, on the one hand, sits uneasily with a core tenet of the concept of cause, on the other. On the leading philosophical and scientific-methodological accounts of causation, a cause is something that makes a difference in conditions that are, broadly speaking, “otherwise equal.” But if race marks social difference, then what is it for two persons or groups that are differently racialized to be “otherwise equal” in the sense required by good causal inquiry into the effects of race?
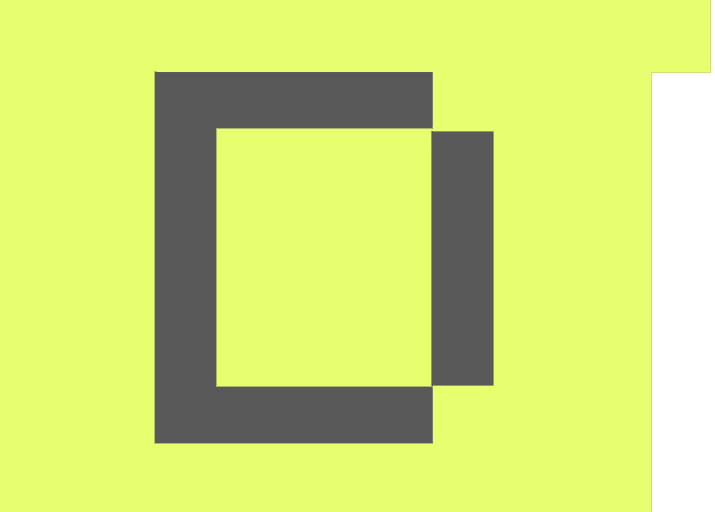
Ela Leshem
Fordham School of Law
Law's Shrinking Circle
The U.S. legal system has historically attributed moral personhood to a wide circle of entities. Among them, for instance, are gods and sovereigns, religious artifacts, sacred sites, national monuments, flags, corpses, and effigies. The law has narrowed this circle of moral concern over time. But its historical personhood conceptions continue to shape many entities’ legal status today. And this legacy holds lessons for contemporary debates over the personhood and property status of natural landscapes, fetuses, AI systems, and more.
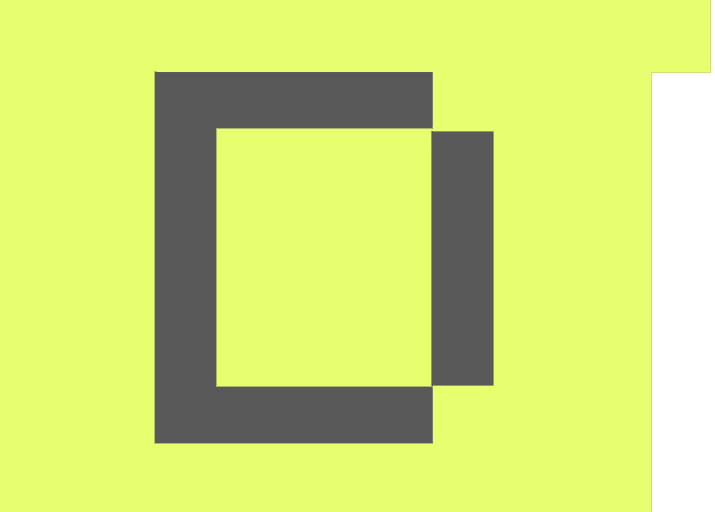
Shahrzad Haddadan
Rutgers University
Optimally Improving Cooperative Learning in a Social Setting
Many important economic and social decisions, beliefs, and attitudes arise from a learning process that
depends on the exchange of imperfect information across a potentially large and complex social network
involving heterogenous and self-interested agents. For example, an individual may seek to determine the veracity of a news story or image of consequence encountered on social media. Towards this end, they may interact with and gain information from other social media users or AI tools – search algorithms, AI-generated social media content, or large-language models.
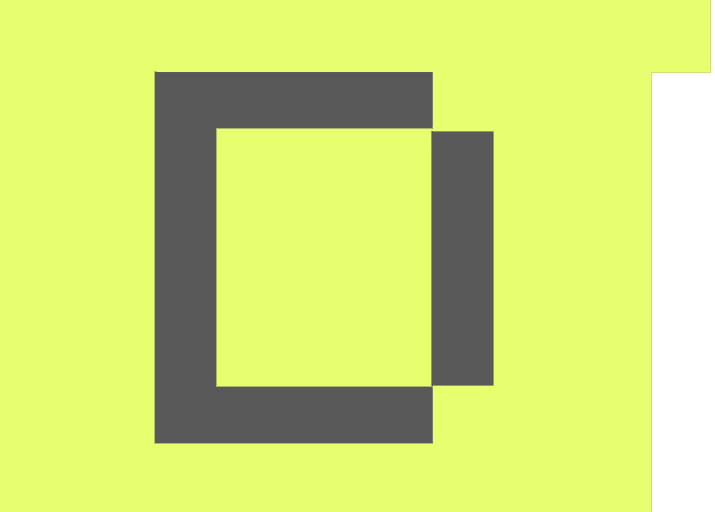
Susannah Glickman
Stony Brook University
The Politics of Exponential Growth: Defense, Technology and Industrial Policy
Discussions of high-technology and its history in the US have often elided the role of the state. This elision is a product of the shifting politics, institutions and infrastructures around this industry but it does not reflect an honest accounting of the historic role of (often military-backed) industrial policy. Military Keynesianism has been a constant, even through the so-called neoliberal era (1975-2016). Various attempts to shift out of this political economy have routinely failed for historically specific reasons that will be examined in the talk. Neoliberal reforms in the 1980s and 1990s spawned a revolt in the tech and defense sectors. This revolt produced both center-left and right iterations of our present politics.
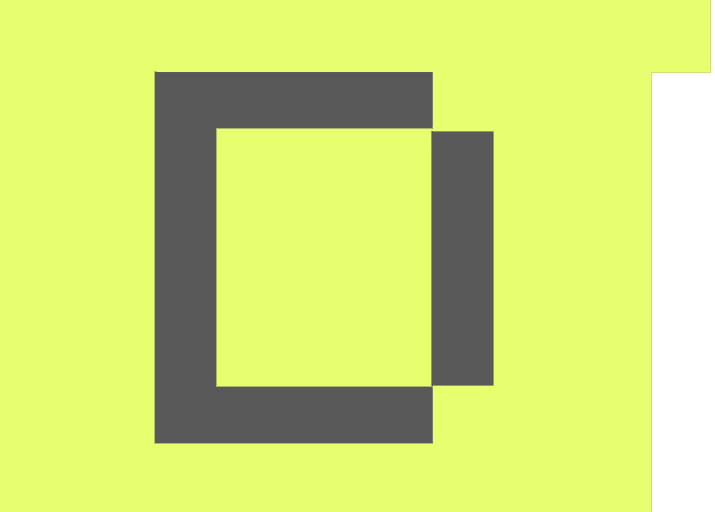
Artur Pericles Lima Monteiro
Yale Law School
Political Constitutionalism for Platforms
Two blueprints for social media reform seemingly present a dilemma: either accept platforms as the rules of the public sphere (and use regulation to tame them) or divest platforms of power by disabling platform governance. That is a false choice, one that sounds like the “great difficulty” the Federalists discussed: protecting people requires government, but people also require protection from the government. This Article draws on the way out of the dilemma that constitutionalism builds on: dividing power.
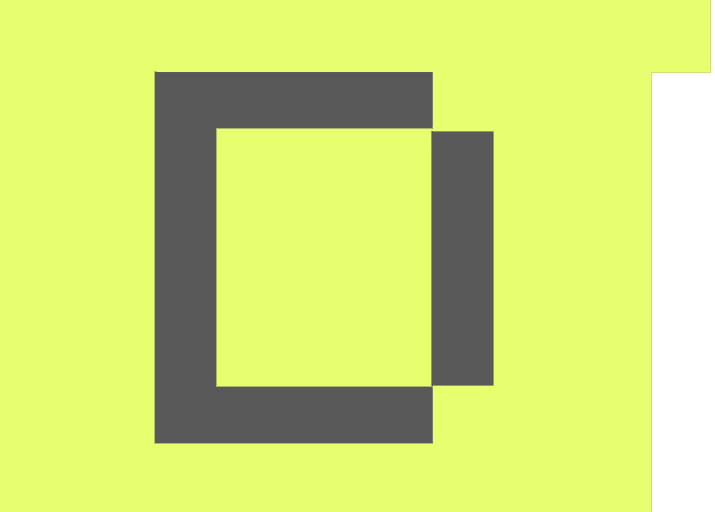
Aniket Kesari
Fordham School of Law
Opinions on Opinions: Legal Reasoning and Court Approval
Judges in common-law systems write detailed opinions not only to explain their decisions to the parties involved, but also as a form of public accountability. Do the legal arguments in these opinions really bolster institutional legitimacy? We analyze this question in the context of legal arguments made by U.S. Supreme Court judges, where we use legal AI to simplify and decompose complex judicial opinions and produce neutral, readable statements of facts and reasoning. In a well-powered pre-registered survey experiment, we ask whether respondents exposed to judicial reasoning change their attitudes toward the Court and its decisions, relative to control-group respondents who view only the facts and the decision (without the legal reasoning).
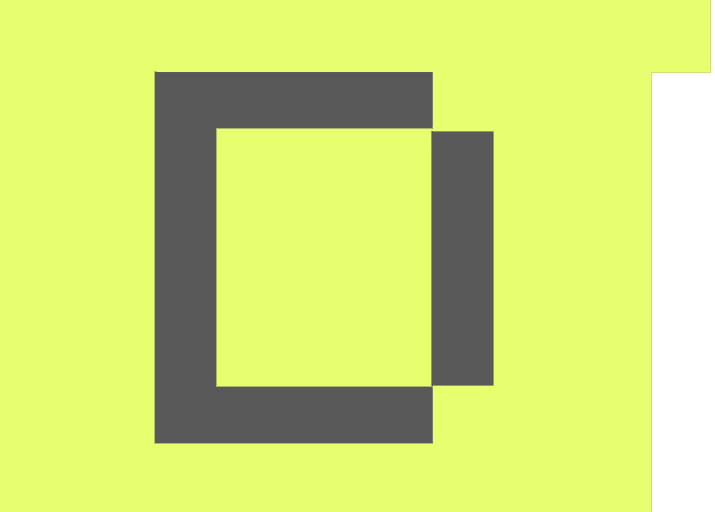
Michael Goodyear
NYU School of Law
Artificial Infringement
Generative AI is changing the way we do everything from legal research to artistic creation. This is possible through recent advances in machine learning that allow AI systems to program themselves. With greater AI capacity, however, comes increasingly unpredictable outputs. AI systems will often generate an output the user and the developer never considered. Sometimes, these unforeseen outputs can infringe others’ copyrights in creative works. In the past two years, copyright law has become one of the leading legal and policy battlegrounds for generative AI. Yet the question of who should be liable when AI systems infringe has barely been addressed.
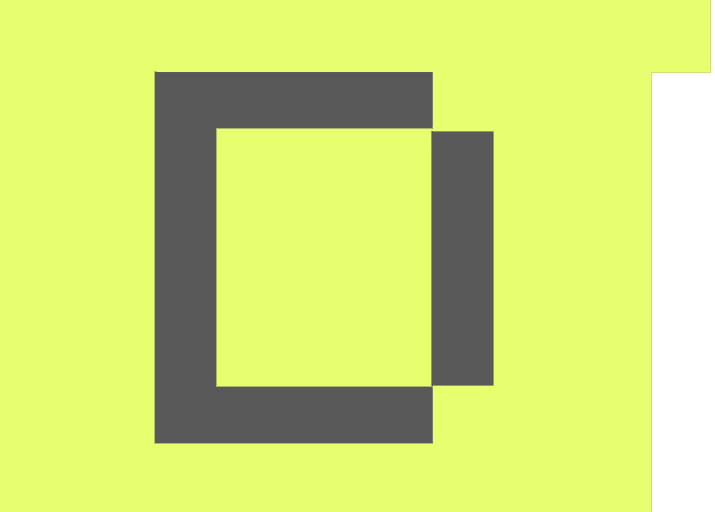
Severin Engelmann
Cornell Tech
A Governance Model for AI Inferences
We are constantly being interpreted, simulated, and evaluated by machines based on the data we generate, both consciously and unconsciously. Even a simple action like picking up a smartphone triggers sensors that collect data, which AI models then leverage to infer aspects of our lives, from our mood to our health. The rapid evolution and decreasing cost of large language models now allow them to predict our responses to surveys or even play economic games on our behalf with surprisingly little input, often achieving remarkable accuracy. In this talk I will ask: What are our possibilities and limits of governing information in an age of AI?
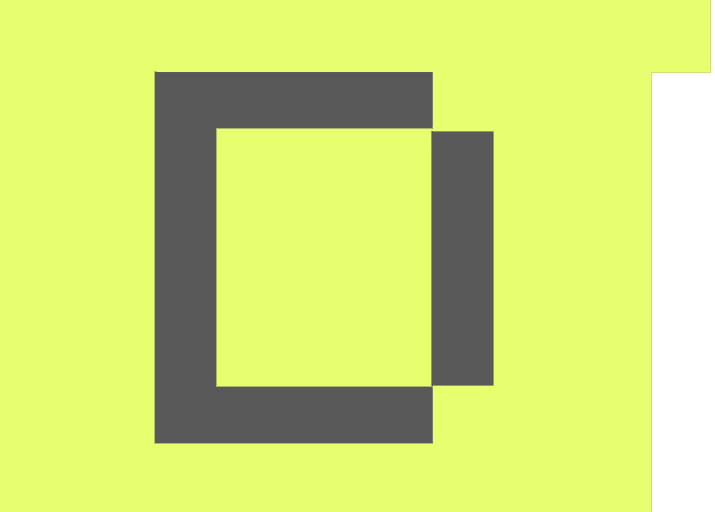
David Gray Widder
Cornell Tech
AI Supply Chains: Tools to Locate Power and Responsibility in AI Production for Critical, Accountable Computing
Contemporary AI production is structured to enable ignorance of harm for those who want it, and complicate resistance for those who don’t. Through case studies of the fractured AI supply chains in a variety of contexts—open source deepfake tools, NASA’s AI autocoder, and AI engineers' own ethical concerns—this talk will examine how a lack of relationality between distributed sites of AI production fractures knowledge, responsibility, and power, leading to unaccountable harm.

Yafit Lev-Aretz
Baruch College, CUNY
Privacy as a Matter of Public Health
State and federal action in privacy law is surging. But much of the new activity is more of the same old: an emphasis on individual choice and narrowly confined rights coupled with silence as to the dispersed, corrosive effects to social welfare that can be brought about by privacy-invasive technology. Privacy law needs a drastic and imaginative shift, and we propose one: understanding privacy as a matter of public health.
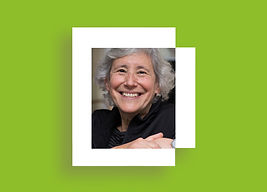
Lucy Suchman
Lancaster University
Disarming the Kill Chain: The Unlawful Imprecision of Algorithmic Warfare
In military parlance ‘the kill chain’ refers to procedures through which persons become targets for the use of lethal force. In the context of war, the legitimacy of killing under International Humanitarian Law turns on the identification of persons who are in combat and pose an imminent threat to the safety of those in whose names weapon systems are deployed. Building on my past work studying the notion of accuracy in the U.S. Department of Defense, and focusing on investments by the DoD and on the Israeli Defense Force’s current operations in the Occupied Palestinian Territories, this talk will question the frames of war that justify the promotion of AI technologies as means of accelerating the operations of targeting.

Sidhika Balachandar & Maya Mundell
Cornell Tech
"Using Graph Neural Networks to Model Biased Crowdsourced Data for Urban Applications" & "Fostering Tech Entrepreneurialism & Entrepreneurial Infrastructure Among Marginalized Communities"
"Using Graph Neural Networks to Model Biased Crowdsourced Data for Urban Applications": Graph neural networks (GNNs) are widely used on graph-structured data in urban spatiotemporal forecasting applications, such as predicting infrastructure problems and weather events. In such settings, nodes have a true latent state (e.g., street condition) that is sparsely observed (e.g., via government inspection ratings). We more frequently observe biased proxies for the latent state (e.g., via crowdsourced reports) that correlate with resident demographics.
"Fostering Tech Entrepreneurialism & Entrepreneurial Infrastructure Among Marginalized": CommunitiesMarginalized entrepreneurs and those at society’s fringes often lack access to entrepreneurial infrastructure such as business education, access to capital, and payment processing. Drawing from several multi-year interview case studies with digital sex workers, influencers, content creators, tech innovators, leaders, startup founders and impact investors, this thesis explores individual, institutional, and philanthropic approaches to addressing the lack of access marginalized populations have to tech entrepreneurship and representation in global startup culture.

Johannes Himmelreich
Syracuse University
Preserving Democracy in the AI-Augmented State: The Role of Responsible Public Service Norms
Democracies augment their bureaucratic decision-making increasingly with artificial intelligence (AI). This AI-augmentation is a threat to democratic governance. And this threat is much deeper than is commonly understood, or so I argue in this paper: The usual metrics to assess AI risks and benefits—i.e., bias, or procedural and substantive fairness—fail to capture the full extent to which the AI-augmented state undermines democracy. Instead, norms of responsible public service offer a more comprehensive basis for assessing AI’s risks and benefits.

Moshe Vardi
Rice University
Lessons from Texas, COVID-19, and the 737 Max: Efficiency vs. Resilience
In both computer science and economics, efficiency is a cherished property. The field of algorithms is almost solely focused on their efficiency. The goal of AI research is to increase efficiency by reducing human labor. In economics, the main advantage of the free market is that it promises “economic efficiency.” A major lesson from many recent disasters is that both fields have overemphasized efficiency and underemphasized resilience.
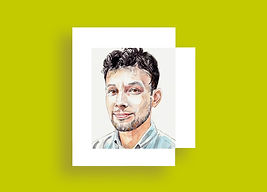
Ben Sobel
Cornell Tech
A Real Account of Deep Fakes
Laws regulating pornographic deepfakes are often characterized as protecting privacy or preventing defamation. But privacy and defamation laws paradigmatically regulate true or false assertions of fact about persons. Anti- deepfakes laws do not: the typical law bans even media that no reasonable observer could understand as factual. Instead of regulating statements of fact, anti-deepfakes laws ban outrageous depictions per se. This is a significant and unrecognized departure from the established dignitary torts, and it is important to acknowledge for two reasons.

Luke Stark
University of Western Ontario
Animation and Artificial Intelligence
Animation increasingly defines the cultural contours of the twenty-first century and is broadly used across many forms of digital media. More than just cartooning, puppetry, or CGI, animation is a paradigm involving the projection of qualities perceived as human such as power, agency, will, and personality outside of the self and onto objects in the environment. In this talk, I argue ChatGPT and similar interactive AI systems, both powered by Large Language Models (LLMs) and not, can be best understood as animated entities.

Julie Cohen
Georgetown Law
Oligarchy, State, and Cryptopia
Influential groups of thinkers, entrepreneurs, and activists argue that networked digital technologies offer potent mechanisms for counteracting and neutralizing state and private power. In particular, they argue that blockchain-based technologies for decentralized, secure authentication of identity and provenance supply the key to unwinding contemporary relations of dominance and replacing them with durably decentralized, bottom-up governance arrangements. This seminar pulls on two interrelated threads in emergent narratives about decentralized digital futures.

Ngozi Okidegbe
Boston University
Rethinking the Place of the Public in Algorithm Governance
The use of artificially intelligent algorithms in local public decision-making is under immense scrutiny. Policymakers, activists, and scholars increasingly question why agencies and courts should have unfettered discretion to deploy privately developed algorithms that affect citizens’ rights, liberties, and opportunities without adequate democratic accountability. In recent years, the injustices faced by those subjected to inaccurate, biased, or procedurally unfair algorithmic predictions have captured the media, igniting a nationwide movement to govern the state’s use of algorithms in the public sphere.

Aleksandra Korolova
Princeton University
Lessons from Auditing the Hidden Societal Impacts of Ad Delivery Algorithms
Although targeted advertising has been touted as a way to give advertisers a choice in who they reach, increasingly, ad delivery algorithms designed by the ad platforms are invisibly refining those choices. In this talk, Aleksandra Korolova will present findings from "black-box" auditing of the role of ad delivery algorithms in shaping who sees opportunity and political ads using only the tools and data accessible to any advertiser. She will then discuss legal and policy efforts to mitigate the harmful effects of ad delivery in these domains, including their shortcomings and potential paths forward.

David Gray Widder
Cornell Tech
Epistemic Power in AI Ethics Labor: Legitimizing Located Complaints
What counts as legitimate AI ethics labor, and consequently, what are the epistemic terms on which AI ethics claims are rendered legitimate? Based on 75 interviews with technologists including researchers, developers, open source contributors, and activists, this talk explores various epistemic bases from which AI ethics is discussed and practiced. In the context of outside attacks on AI ethics as an impediment to “progress,” David Widder shows how some AI ethics practices have reached toward scholarly authority, automation and quantification and achieved some legitimacy as a result, while those based on richly embodied and situated lived experience have not.

Jake Goldenfein
Melbourne Law School
Untangling the Loop – Four Legal Approaches to Human Oversight
As automation and AI re-organise social, political, and economic life, law’s reflex is often towards the human. By ensuring automation is “human-centred” and subject to “human oversight”, the hope is that automation’s economic promises can be safely realised. Despite the intuitive appeal, analysts have started documenting the empirical failure of human oversight to improve decision quality. But this creates a conundrum. There may be little scientific evidence supporting legal human oversight requirements, but abdicating human agency in automated decision processes is ethically and politically unfeasible.

Frank Pasquale
Cornell Tech & Cornell Law School
Data Access & AI Explainability
Several jurisdictions are now expanding their data protection laws in response to proliferating AI-driven evaluations of consumers, workers, borrowers, and internet users. As they digitize judgment, these evaluations risk imposing benefits and burdens in opaque and unaccountable ways via automated decision-making. Information access rights guaranteed via data protection law can assist those who have been treated unfairly—but only if they are clarified and enforced well. The key to doing so, Pasquale claims, is close attention to the practical consequences of data access and explainability.

Hauke Sandhaus & Ian René Solano-Kamaiko
Cornell Tech
"Bright Patterns: Towards Extra Ethical User Experience Design" & "Explorable Explainable AI: Improving AI Understanding for Community Health Workers in India"
"Bright Patterns: Towards Extra Ethical User Experience Design": Dark Patterns have received significant attention from the academic Human-Computer Interaction community since 2010, when Brignull launched darkpatterns.org and initially described them as "tricks used in websites and apps that make you do things that you didn’t mean to." After years of collective action, governments worldwide are recognizing the need to regulate them—with the GDPR in the EU, the FTC in the U.S., and, more recently, the CCPA in India, all prohibiting specific types of Dark Patterns. – Hauke Sandhaus
"Explorable Explainable AI: Improving AI Understanding for Community Health Workers in India": AI technologies are increasingly deployed to support community health workers (CHWs) in high-stakes healthcare settings, from malnutrition diagnosis to diabetic retinopathy. Yet, little is known about how such technologies are understood by CHWs with low digital literacy and what can be done to make AI more understandable for them. – Ian René Solano-Kamaiko
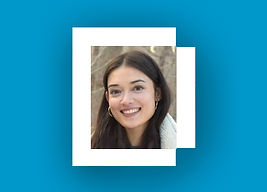
Kat Geddes
Cornell Tech & NYU
Protecting Amateur Creativity in the Age of Generative AI
The advent of text-to-image models that can produce sophisticated digital images in a matter of seconds has raised alarm bells within the artistic community. Human artists working with more traditional media have warned about the effects of generative models on the market for their works, and the future of human creativity. While artists whose works were involuntarily used to train generative models have legitimate cause for complaint (and reasonable demands for compensation and attribution), the emergence of generative models is not as apocalyptic as framed. By providing amateur creators with powerful tools for creative expression, generative models help to democratize cultural participation, and diversify public discourse. Accordingly, those who view human creativity as a relational and dialogic practice should embrace generative AI’s capacity to extend this practice to previously excluded communities.
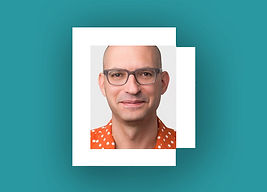
Mor Naaman
Cornell Tech
AI and the Future of Human Communication
From autocomplete and smart replies to video filters and deepfakes, we increasingly live in a world where communication between humans is augmented by artificial intelligence. AI often operates on behalf of a human communicator by recommending, suggesting, modifying, or generating messages to accomplish communication goals. We call this phenomenon AI-Mediated Communication (or AI-MC). While AI-MC has the potential of making human communication more efficient, it impacts other aspects of our communication in ways that are not yet well understood. Over the last six years, my collaborators and I have been documenting the impact of AI-MC on communication outcomes, language use, interpersonal trust, and more. The talk will outline experimental findings from this work.
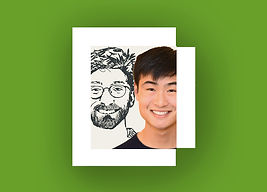
Amritansh Kwatra & Kenny Peng
Cornell Tech
"Asynchronous Workflows for Maintaining Hardware" &
"Algorithmic Monoculture: Modeling Risks and Benefits"
"Asynchronous Workflows for Maintaining Hardware": Hardware is challenging for end-users to troubleshoot, repair, and maintain on their own. As a result, they seek out expert technicians to remedy their issues. This kind of synchronous support is limited by expert availability and is challenging to scale. DLI Doctoral Fellow Amritansh Kwatra will introduce SplatOverflow, a workflow that enables asynchronous maintenance of hardware; allowing experts to assist end-users without the need for live communication.
"Algorithmic Monoculture: Modeling Risks and Benefits": More than half of the 100 largest companies in the U.S. now use HireVue's screening algorithms. What are the risks and (perhaps) benefits of an emerging algorithmic monoculture, where many decision-makers rely on the same algorithm to make consequential decisions? In this talk, DLI Doctoral Fellow Kenny Peng will present findings from a new mathematical model of algorithmic monoculture, challenging some prevailing intuitions.
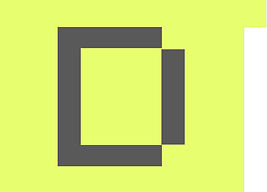
Ben Sobel
Cornell Tech
Copyright Accelerationism
Modern copyright law seems determined to impede people’s engagement with creative expression. Rock-bottom creativity requirements, the abolition of formal prerequisites to copyright ownership, and an irrationally long copyright term ensure that nearly all recorded culture is encumbered not merely for years, but for generations. Today, however, change is in the air—for all the wrong reasons. By historical accident, the same foundational properties of copyright law that have long undermined creators and audiences now pose an existential threat to generative AI. Tech companies and their allies are pushing to reform the very aspects of copyright law that impoverish traditional readership and authorship. But by and large, their proposals would change these doctrines only in ways that benefit the generative AI enterprise. This essay offers an alternative: copyright accelerationism.
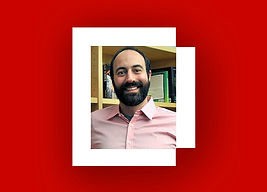
Ryan Calo
University of Washington Law School
Socio-Digital Vulnerability
Social technologies such as chat, smart speakers, and personal robots highlight the growing concern and nuance around the safety and privacy of vulnerable populations in mediated environments. In this paper, we first critique the way law treats vulnerability as binary or status based. Next, drawing from various phenomena and literature such as dark patterns, digital market manipulation, and computers as social actors, we develop a theory of socio-digital vulnerability.
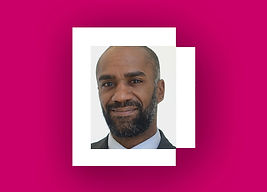
Olivier Sylvain
Fordham University Law School
Platform User Rights Are No Rights at All
Recent policy developments suggest that consumer sovereignty models of regulation have substantial, if not fatal, limitations. Binding decisions by the European Data Protection Board in 2023, as well as other recent public law enactments in the EU and the US, overtly reject the assumption that individuals are best situated to manage how companies process or use their personal information.
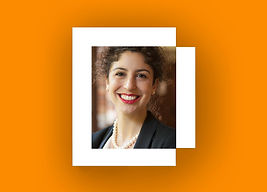
Rebecca Wexler
UC Berkeley School of Law
Police Secrecy: Law Enforcement Privilege and the Criminally Accused
You can’t question a secret you haven’t been told. The criminal legal system depends on fair and open proceedings to expose and regulate unlawful and unconstitutional police conduct through the courts. If police can use claims of secrecy to systematically thwart criminal defendants' access to evidence, judicial review will fail. And yet that is exactly what is happening under a common-law doctrine called the “law enforcement privilege.” The privilege empowers police and prosecutors to rely on the results of secret investigative methods while withholding information from the defense about how those methods work.

Benjamin Mako Hill
University of Washington
Lifecycles of Peer-produced Knowledge Commons
After increasing rapidly over seven years, the number of active contributors to English Wikipedia peaked in 2007 and has been in decline since. I will present a body of evidence that suggests that English Wikipedia's pattern of growth and decline appears to be a general feature of "peer production"—the model of collaborative production that has produced millions of wikis, free/open source software projects, websites like OpenStreetMap, and more.

Sarah M. Brown
University of Rhode Island
Designing a Tool to Measure Perceptions of AI Fairness
Understanding the impact of AI on society requires understanding how people feel about their impact and what people, outside those building them, think AI should do. However, while performance of algorithms is measured in terms of a now field-standard set of performance metrics, these metrics and their interpretation are not standard knowledge for most people who are impacted by these systems. To express their thoughts reliably, non-expert study participants need to understand what they are being asked.
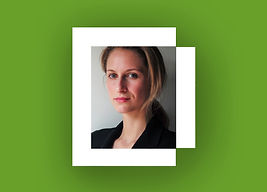
Zoë Hitzig
Harvard University
"Equity" and "Privacy" in Mechanism Design
I will begin with an overview of mechanism design, the field of microeconomic theory focused on the design of institutions and rules that produce good social outcomes. I will discuss a prominent case in which mechanism design was used to advocate for a major policy change in the name of equity – a change in the algorithms used to assign K-12 students to public school in Boston. I will talk about how, inevitably, a gap emerges between what the theory suggests, and what stakeholders think the theory suggests.

Julian Thomas & Jean Burgess
Australian Research Council Centre of Excellence for Automated Decision-Making and Society
Society in the Loop: Observability and Explainability in Automated News and Media
This talk outlines the general approach of the ADM+S Centre to issues of automated decision-making in the news and media domain, addressing both the challenges for researchers seeking to understand developments in this field, and the questions of policy, ethics and regulation that arise from it. We use this lens to introduce several research projects underway in the Centre, focussing on observability and explainability in relation to key applications and infrastructures (search engines), business systems (social media advertising), and the social distribution of human capabilities (digital inclusion among vulnerable communities).
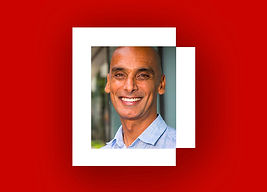
Salil Vadhan
Harvard University
OpenDP: A Community Effort to Advance the Practice of Differential Privacy
Since it was introduced in 2006 by theoretical computer scientists Dwork, McSherry, Nissim, and Smith, differential privacy has become the leading framework for ensuring that individual-level information is not leaked through statistical releases or machine learning models built from sensitive datasets. In addition to a rich theoretical literature, differential privacy has also started to make the transition to practice, with large-scale applications by the US Census Bureau and technology companies like Google, Apple, Microsoft, and Meta.
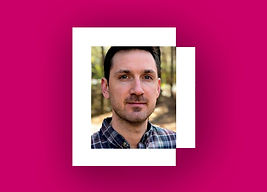
Lee McGuigan
University of North Carolina, Chapel Hill
Selling the American People: Advertising, Optimization, and the Origins of Adtech
Algorithms, data extraction, digital marketers monetizing "eyeballs": these all seem like such recent features of our lives. And yet, Lee McGuigan tells us in this eye-opening book, digital advertising was well underway before the widespread use of the Internet. Explaining how marketers have brandished the tools of automation and management science to exploit new profit opportunities, Selling the American People traces data-driven surveillance all the way back to the 1950s, when the computerization of the advertising business began to blend science, technology, and calculative cultures in an ideology of optimization. With that ideology came adtech, a major infrastructure of digital capitalism.
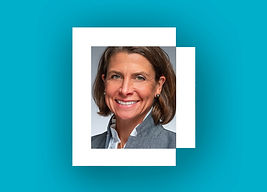
Kirsten Martin, Helen Nissenbaum & Vitaly Shmatikov
University of Notre Dame and Cornell Tech
No Cookies for You: Evaluating the Promises of Big Tech’s “Privacy-Enhancing” Techniques
We examine three common principles underlying a slew of “privacy-enhancing” techniques recently deployed or scheduled for deployment by big tech companies: (1) “We deny (or throttle) access to your data by third parties!”; (2) “We minimize the use and retention of raw data!”; and (3) “Your data never leaves your device!” Our article challenges these principles, not on the grounds that techniques offered to implement them are unsuccessful in achieving their stated goals. Instead, we argue that the principles themselves fall short because their underlying conception of privacy is flawed.

Severin Engelmann
Cornell Tech
Effectively Countering Hate Speech on X
Effectively reducing hate speech on social media is a defining challenge of the digital age. Hate speech expressions inflict non-trivial harm to individuals or groups based on their ethnicity, gender, religion and other characteristics. Hate speech’s lasting effects silence and marginalize vulnerable communities. When people see hate speech on social media they sometimes counter it publicly by condemning the transgression itself and/or by showing solidarity with the victim. In an in-the-wild study on X (formerly Twitter), we controlled user accounts to deliberately counter racist slurs and investigated whether transgressors would change their transgression behavior following our intervention.

Madiha Zahrah Choksi
Cornell Tech
How Licenses Learn
Open-source licenses are infrastructure that collaborative communities in-habit. These licenses don’t just define the legal terms under which members (and outsiders) can use and build on the contributions of others. They also reflect a community’s consensus on the reciprocal obligations that define it as a community. A license is a statement of values, in legally executable form, adapted for daily use. As such, a license must be designed, much as the software and hardware that open-source developers create. Sometimes an existing license is fit to purpose and can be adopted without extensive discussion. However, often the technical or social needs of a community do not precisely map onto existing licenses, or the community itself is divided about the norms a license should enforce.
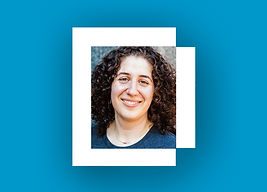
Josephine Wolff
Tufts University
Lessons Lost: How Lawyers Undermine Cybersecurity Investigations
Lawyers lead the investigations for many cybersecurity incidents, ranging from data breaches to ransomware, in part because they can often shield any materials produced after a breach from discovery under either attorney-client privilege or work product immunity. Moreover, by limiting and shaping the documentation that is produced by breached firms’ personnel and third-party consultants in the wake of a cyberattack, attorneys can limit the availability of potentially damaging information to plaintiffs’ attorneys, regulators, or media, even if their attorney-client privilege and work product immunity arguments falter.

Sina Fazelpour
Northeastern University
Fairness in Sociotechnical Machine Learning Systems
Machine learning (ML) algorithms play an increasingly prominent role in the distribution of benefits and burdens in sensitive domains. However, ML systems risk introducing biases that undermine values of justices and fairness, for instance, by perpetuating or even exacerbating unjustifiable harms against vulnerable communities. In response to this concern, a burgeoning field of fair ML has emerged, with researchers developing various fairness measures and methodologies for quantifying and mitigating algorithmic harms. From this perspective, philosophical theorizing about ML tools is often constrained to clarifying the normative underpinnings of fairness measures to help resolve disagreements arising from impossibility results and formal trade-offs. In this talk, Sina Fazelpour argue that this type of fair ML strategy is usefully characterized as a problematic form of ideal theorizing about justice, and thus suffers from limitations known to plague that approach more broadly.

Smitha Milli
Cornell Tech
Experimentally Measuring Effects of Recommender Systems
As social media continues to have a significant influence on public opinion, understanding the impact of the machine learning algorithms that filter and curate content is crucial. However, existing studies have yielded inconsistent results, potentially due to limitations such as reliance on observational methods, use of simulated rather than real users, restriction to specific types of content, or internal access requirements that may create conflicts of interest. To overcome these issues, we conducted a pre-registered controlled experiment on Twitter's algorithm without internal access. The key to our design was to, for a large group of active Twitter users, simultaneously collect (a) the tweets the algorithm shows, and (b) the tweets the user would have seen if they were just shown the latest tweets from people they follow; we then surveyed users about both sets of tweets in a random order.

Ero Balsa
Cornell Tech
The Risks of Privacy Risk Assessments
Privacy risk assessments have been touted as an objective, principled way to encourage organizations to implement privacy-by-design. They are central to a new regulatory model of collaborative governance, as embodied by the GDPR. However, existing guidelines and methods are vague, and there is little empirical evidence on privacy harms, questioning the suitability of privacy risk assessments as an effective policy instrument. In this talk, Ero Balsa will present a close analysis of US NIST’s Privacy Risk Assessment Methodology, highlighting multiple sites of discretion that create countless opportunities for adversarial organizations to engage in performative compliance.
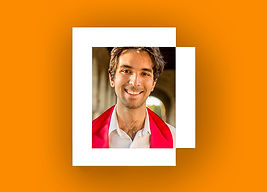
Armin Namavari
Cornell Tech
Governance for End-to-End Encrypted Communities
The increasing harms caused by hate, harassment, and other forms of abuse online have motivated major platforms to explore hierarchical governance. The idea is to allow communities to have designated members take on moderation and leadership duties; meanwhile, members can still escalate issues to the platform. But these promising approaches have only been explored in plaintext settings where community content is public to the platform. It is unclear how one can realize hierarchical governance in the huge and increasing number of online communities that utilize end-to-end encrypted (E2EE) messaging for privacy.

Aileen Nielsen
ETH Zurich
The Too Accurate Algorithm
Much research on the law and policy of algorithms has focused on ways to detect or prevent algorithmic misbehavior or mistake. However, there are also problems that result when algorithms perform their assigned tasks too well rather than too poorly. This presentation makes the case that significant individual harms and social welfare losses alike can and do occur in the face of the ever more common phenomenon of the too accurate algorithm.
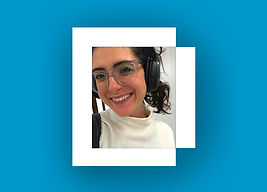
Margot Hanley
Cornell Tech
Producing Personhood: How Designers Perceive and Program Voice Assistant Devices
Artificial intelligence has become increasingly ingrained in the fabric of everyday life, yet sociologists know little about how technology producers design artificial intelligence. Margot will present her research which draws upon in-depth interviews with twenty-one voice assistant designers at major technology companies. The study examines how engineers weighed multiple and sometimes competing organizational goals in making decisions about how to produce “personhood” in voice assistant devices.
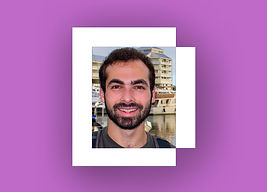
Dan Adler
Cornell Tech
Mental Health Digital Biomarkers: Moving from Research to Practice
Mental health "digital biomarkers" purport to measure mental health and well-being using data collected from mobile devices and technology platforms (eg, location, usage patterns). Translating digital biomarkers into clinical practice raises multiple sociotechnical questions that need to be addressed if these computational tools intend to improve care. How do we assess whether digital biomarkers will be reliable in clinical settings, and even in cases where measurement is reliable, will digital biomarkers improve mental health?
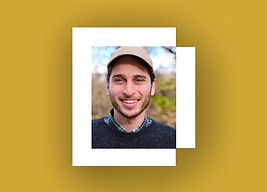
Ben Laufer
Cornell Tech
What is "Algorithmic Amplification" and when is it wrongful?
Increasingly concerned about the way in which content spreads on the internet, scholars reach for the concept of "algorithmic amplification" (AA) as both an explanation and a warning. Although these researchers frequently acknowledge the metaphorical and conceptual haziness around the term, they continue to rely on it to carry both descriptive and normative intent. This project aims to do the foundational work to give "algorithmic amplification" conceptual precision and normative teeth.

Lior Zalmanson
Cornell Tech
When Algorithms Are Your Boss: The Anatomy of Algorithmic Management
When humans first imagined robots and computers in the workplace, they envisioned them as servants or supporters of humankind. However, the integration of technology in the workplace has evolved. In recent years, numerous online platforms, such as Uber or Doordash, have been "employing" large numbers of human workers for tasks supervised, controlled, and managed by algorithms - a phenomenon known as algorithmic management. This shift in the role of technology raises several critical questions, such as how it feels to have an AI algorithm as a boss and how human workers react to maltreatment by algorithms.
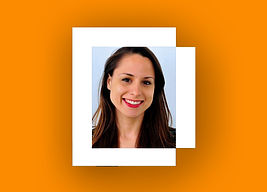
Robyn Caplan
Cornell Tech
Taking Back and Giving Back: Redistributing Value in the Algorithmic Economy
Research on algorithmic imaginaries related to creators and influencers often focuses on their efforts to understand and best navigate algorithms to maximize visibility, and thus profits. However, there has been less work on how non-influencers work together to redistribute algorithmically-produced visibility through beliefs about how algorithms ought to work. Using "algorithmic ethnography" (Christin, 2020), Caplan and her co-authors, Elena Maris (UIC) and Hibby Thach (UIC) have identified three TikTok genres that they argue are emblematic of how practices of mutual aid (Spade, 2020) are unfolding over platforms.

Elettra Bietti
Cornell Tech
From Data to Attention: Regulating Extraction in the Attention Platform Economy
Rethinking the regulation of advertising-based platform business models such as Facebook/Meta and Google/Alphabet, which I call attention platforms, is an urgent task. Two decades of regulatory apathy and intellectual fragmentation have produced siloed approaches to the regulation of data and content that leave many urgent political, economic and environmental issues unaddressed. In this paper, I argue that current approaches to regulating data and datafication – approaches that regulate control over personal data or that focus on regulating social data – fail to address the most pervasive forms of extraction and harm in the attention platform economy: those that stem from addiction, over-consumption, virality, and fragmentation of the public sphere.
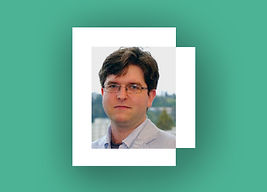
Thomas Krendl Gilbert
Cornell Tech
Are We All Miasmists Now? Parallels Between Recommender Systems and the History of Public Health
Attention capitalism has generated design processes and product development decisions that prioritize platform growth over all other considerations. To the extent limits have been placed on these incentives, interventions have primarily taken the form of content moderation. While moderation is important for what we call “acute harms,” societal-scale harms – such as negative effects on mental health and social trust – require new forms of institutional transparency and scientific investigation, which we group under the name accountability infrastructure.

Michal Gal
Center for Law and Technology, University of Haifa
Synthetic Data: Competitive and Human Dignity Implications
A data-generation revolution is underway. Up until recently, most of the data used for decision-making was collected from events that take place in the physical world ("real" data). Yet, it is forecasted that by 2024, 60% of data used to train artificial intelligence systems around the world will be synthetic (!). Synthetic data is artificially-generated data that has analytical value. For some purposes, synthetic datasets can replace real data by preserving or mimicking their properties. For some others it can complement real data in a way which increases their accuracy or their privacy protection. The importance of this data revolution for our economies and societies cannot be over-stated. It affects data access and data flows, potentially changing the competitive dynamics in markets where real data is not easily collected, and potentially affecting decision-making functions for many spheres of our lives.
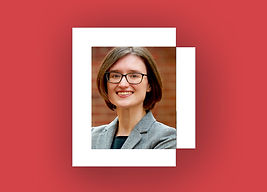
Erin Miller
University of Southern California, Gould Law School
Quasi-State Action in First Amendment Theory
In this talk, Erin Miller will challenge the First Amendment orthodoxy that speech rights bind only the state. She will argue that the primary justification for the freedom of speech is to protect interests like autonomy, democracy, and knowledge from the kind of extraordinary power available to the state. If so, it applies with nearly equal force to any private agents with power over speech rivaling that of the state. Such a class of private agents, which she calls quasi-state agents, turns out to be a live possibility once we recognize that state power is more limited than it seems and can be broken down into multiple, equally threatening parts. They might include, for example, the largest social media platforms and powerful private employers.
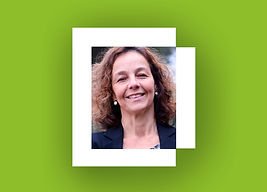
Niva Elkin-Koren
Tel Aviv University
The By-design Approach Revisited: Lessons from Covid-19 Contact Tracing App
Niva Elkin-Koren is a Professor of Law at Tel-Aviv University Faculty of Law and a Faculty Associate at the Berkman Klein Center for Internet & Society at Harvard University. She is a former Dean of University of Haifa Faculty of Law, and the founding director of the Center for Cyber, Law and Policy (CCLP) and of the Haifa Center for Law & Technology (HCLT).

Seth Lazar
Australian National University
Governing the Algorithmic City
A century ago, John Dewey observed that '[s]team and electricity have done more to alter the conditions under which men associate together than all the agencies which affected human relationships before our time'. In the last few decades, computing technologies have had a similar effect. Political philosophy's central task is to help us decide how to live together, by analysing our social relations, diagnosing their failings, and articulating ideals to guide their revision. But these profound social changes have left scarcely a dent in the model of social relations that most (analytical) political philosophers assume.

Judith Simon
Universität Hamburg
Dis/Trusting AI?
In this talk, Judith Simon will first turn to the question whether we can sensibly talk about trust in AI systems. Proposing a socio-technical view on AI, she will argue that we can trust AI systems, if we conceive them as systems consisting of networks of technologies and human actors, but that we should trust them if and only if they are trustworthy. Simon will conclude her talk by outlining some epistemic and ethical requirements for trustworthy systems and two caveats.

Kathleen Creel
Northeastern University
Picking on the Same Person: The Ethics of Algorithmic Monoculture
Human mistakes are inevitable, but fortunately heterogenous. Not so with machine decision-making. Using the same machine learning model for high-stakes decisions in many settings amplifies the strengths, weaknesses, biases, and idiosyncrasies of the original model. When the same person re-encounters the same model again and again, or models trained on the same dataset, she might be wrongly rejected again and again.
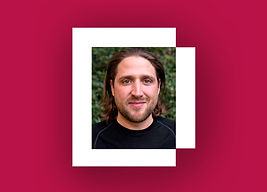
John Basl
Northeastern University
What We Owe to Decision-subjects: Beyond Transparency and Explanation in Automated Decision-Making
In this paper, we defend what we call the Interpretability Thesis which states that, in many contexts, decision-makers are morally obligated to avoid basing their decisions about how to treat decision-subjects on the outputs of non-interpretable ("black box") algorithmic decision systems. Others have defended this thesis, typically by arguing that we have duties of transparency to decision-subjects which require us to make certain information available to them. However, this approach to defending the interpretability thesis has been met with skepticism with skeptics worrying about the grounds of these duties of transparency and concerned that we hold algorithmic decisions to higher standards than human decision systems which also fail to meet duties of transparency.

Daniel Susser
Penn State’s College of Information Sciences and Technology
Exploitation and Platform Power
Big tech “exploits” us. This has become a common refrain among critics of digital platforms. It gives voice to a shared sense that technology firms are somehow mistreating people—taking advantage of us, extracting from us—in a way that other data-driven harms, such as surveillance and algorithmic bias, fail to capture. But what does “exploitation” entail, exactly, and how do platforms perpetrate it? What would a theory of digital exploitation add to existing discussions about platform governance?

Meg Young
Cornell Tech
Data Ownership is Not Dispositive: Data Access Conflicts in Public-Private Contracting Relationships
When firms contract with government agencies to provide services, they regularly assert that some subset of their work is proprietary and confidential. At the same time, public agencies are also subject to transparency requirements. Within the State of Washington, agencies are subject to a strongly transparent Public Records Act, the state's freedom of information law, under which members of the public are granted access to a large share of government information by request. Public agencies also seek access to firms' data to advance accountability, equity, and oversight objectives. In both respects, data access is constrained in practice when firms assert it to be trade secret. Specifically, I analyze two public-private data sharing relationships as a site of contestation over data access and control.

Andre Esteva
Medical AI, Salesforce Research
Frontiers of Medical AI: Therapeutics and Workflows
As the artificial intelligence and deep learning revolutions have swept over a number of industries, medicine has stood out as a prime area for beneficial innovation. The maturation of key areas of AI – computer vision, natural language processing, etc. – have led to their successive adoption in certain application areas of medicine. The field has seen thousands of researchers and companies begin pioneering new and creative ways of benefiting healthcare with AI. Here we’ll discuss two vitally important areas – therapeutics, and workflows.
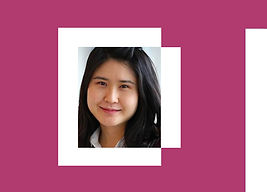
Amy B.Z. Zhang
Cornell Tech
Personalized Recommender Systems: Technological Impact and Concerns
Most of our online activities are, at least in part, powered by personalized recommender systems. While automatic pattern extraction as a technology holds great promise, it can also have alarming adverse impacts. This talk will give a high-level overview of common techniques for personalized recommender systems, and how they connect to problems on both the personal and social level. It will also discuss some alternative approaches addressing these issues, and why a solution cannot come from technology alone.

Salomé Viljoen
Cornell Tech | NYU
The Great Regulatory Dodge
This talk will feature some preliminary thoughts regarding how the current regulatory paradigm in privacy law enables digital technology companies to “dodge” privacy regulations with which other companies offering similar services must comply, and exploring how this “dodge” results in unfair rules for companies and undermines privacy protection for people. Analyzing how the current privacy regime facilitates the dodge is important for diagnosing the shortcomings of existing laws, for revealing harmful effects on individuals and social institutions, and for developing effective alternatives. Such diagnosis gains urgency in light of the growing scholarly and policymaker consensus around privacy law reform.

Ero Balsa
Cornell Tech
Privacy Engineering Through Obfuscation
Privacy engineering seeks to provide tools and methods to design privacy-preserving systems or patch privacy invasive ones. Obfuscation is one of the essential tools in the privacy engineering toolkit. But what can we learn from the plethora of methods and techniques that one may categorize as obfuscation? What can we learn from the role obfuscation plays in privacy engineering? In this talk, Ero Balsa will provide an overview of the two main reasons why privacy engineers resort to obfuscation: to enable people to protect themselves against unnecessarily privacy-invasive systems, and to modulate the level of exposure that providing utility to untrusted parties requires.
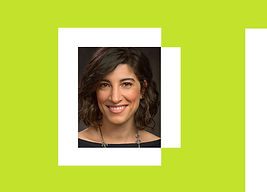
Renée DiResta
Stanford Internet Observatory
From Civics to COVID: Dynamics of Misinformation
From July to January of 2020, Stanford Internet Observatory researchers worked with a coalition of researchers, government entities, tech companies, and civil society organizations in a multi-stakeholder partnership called the Election Integrity Partnership (EIP). Its mission was to rapidly detect high-velocity and potentially impactful false and misleading narratives related to voting. This talk will discuss findings from the partnership: how incidents became narratives, the rise of bottom-up misinformation, the dynamics of repeat spreaders, and the way in which platform policies shape message propagation.

Yan Ji
Cornell Tech
Proof of Liabilities
Cryptographic proof of liabilities (PoL) is a cryptographic primitive to prove the size of funds a bank owes to its customers in a decentralized manner and can be used for solvency audits with better privacy guarantees. Most PoL schemes follow the same principle, i.e., a prover aggregates all of the user balances and enables users to verify balance inclusion in the reported total amount. This process is probabilistic and the more the users who verify inclusion the better the guarantee of a non-cheating prover. In this presentation, Yan Ji introduces generalized PoL, which was originally proposed for proving financial solvency, by extending the state-of-the-art PoL scheme with extra privacy features, and making it applicable to domains outside finance, including transparent and private donations, new algorithms for disapproval voting and negative reviews, and publicly verifiable COVID-19 cases.

Ari Waldman
Northeastern University
Misinformation and the Conservative as Victim
This is an early-stage project about misinformation. While legal scholars have been active over the last 4 years identifying legal definitions of and developing legal responses to the problem of misinformation, including assessing the constitutionality of those responses under the current Supreme Court's First Amendment jurisprudence, less attention has been paid to how the law is already changing as a result of misinformation and how current legal doctrines and institutions are vulnerable to erosion because of misinformation already in the mix. This project brings together literature in sociology and social network theory about how information spreads and doctrinal standards used in judicial review of government action.
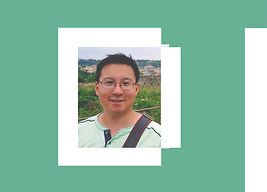
Anthony Poon
Cornell Tech
Thinking Backwards from Improvement in Information Technology Action Research
In information technology for development and related fields, action-oriented researchers aim to design and evaluate how technology can be used to improve the lives of underserved populations around the globe. However, improvement is a value-laden concept with normative, causal, and methodological assumptions. There are many alternative definitions that can be difficult to engage with and tempting for an action researcher to ignore. However, these definitions can heavily influence the direction, design, and evaluation of such work. In this presentation, Anthony Poon discusses some potential perspectives on improvement, including human development, empowerment, and post-development, and how they have influenced some of his past and current work.

John W. Etchemendy (Moderator)
Stanford University
Debate: "Does AI Pose an Existential Threat to Humanity?"
DLI's inaugural debate was inspired by thinking through the provocations posed by the impact of ‘intelligent’ technologies on the future of human life. Will robots take over the planet? Will they undermine or erode what it means to be human in other more subtle or unanticipated ways? Is the preoccupation with intelligent machines a red herring? Or is the biggest threat posed by intelligent machines the affordances they provide to the humans who wield them?

Julia Stoyanovich
New York University
The Unbearable Lightness of Teaching Responsible Data Science
Although an increasing number of ethical data science and AI courses is available, pedagogical approaches used in these courses rely exclusively on texts rather than on algorithmic development or data analysis. Technical students often consider these courses unimportant and a distraction from the “real” material. To develop instructional materials and methodologies that are thoughtful and engaging, we must strive for balance: between texts and coding, between critique and solution, and between cutting-edge research and practical applicability. In this talk, Julia Stoyanovich will discuss responsible data science courses that she has been developing and teaching to technical students at New York University since 2019, and will also speak to some ongoing work on teaching responsible data science to members of the public in a peer learning setting.

Mary Flanagan
Dartmouth College
Games as Social Transformation
Can games make the world a better place? Is it possible that we use games to make a difference in global challenges such as climate change or public health? Can we reduce societal biases, or encourage people to intervene in situations of danger, such as sexual assault? And how do we know the games are doing what they set out to do?
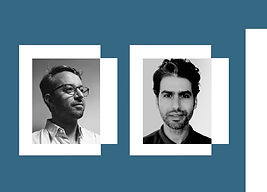
Robin Berjon & Ido Sivan-Sevilla
New York Times | Cornell Tech
AdTech & Our Privacy – Dark present, brighter future?
This joint session is about the digital advertising ecosystem: we highlight some of its disturbing practices against users’ privacy, explain the puzzle of lack of GDPR enforcement over its clear data protection violations, provide a glimpse on how a major publisher with a significant ad operation, The New York Times, has been trying to safeguard the privacy of its readers without forgoing revenue, and conclude by looking ahead at the current conversations in the web standards community on how to build an ad ecosystem without ubiquitous tracking.
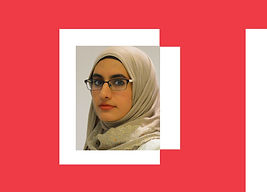
Samar Sabie
Cornell Tech
Is Unmaking Design?
Design does more than supply the market with new products and services; it can raise provocations, critique existing socio-technical arrangements, seed conversations around matters of concern, and imagine radical alternatives. However, even when design is used as a critical provocation or political contestation, the focus is often on ‘making’ something new - a product, interface or artifact. That is because ‘unmaking’, a natural aspect of the designerly transformations always underway in the worlds around us, remains invisibilized and rarely theorized as its own explicit and intentional strategy.
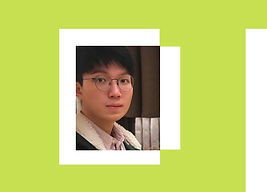
Congzheng Song
Cornell Tech
Measuring the Unmeasured: New Threats to Machine Learning Systems
Machine learning (ML) is at the core of many Internet services and operates on users’ personal information. The deciding metric for deploying ML models is often test performance, which measures if the models learned the given task well. Test performance, however, does not measure other important properties of ML models such as security vulnerabilities, privacy leakage and compliance with regulations.

Gary Johnson, Molly Turner & Ren Yee
Panel Discussion
The Platform Insurgency: Does Urban Tech Have an Ethics Problem?
Much of urban tech exploits today’s most ethically-charged technologies and business practices—such as indiscriminate location tracking, facial recognition, and gig work to fundamentally reprogram how urban systems function. As these failures become clearer, and broader awareness of systemic injustice in society grows, how can the emerging field of urban tech clarify choices between right and wrong?
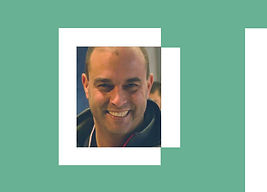
Joshua A. Tucker
New York University
The Truth About Fake News: Measuring Vulnerability to Fake News Online
How well can ordinary people do in identifying the veracity of news in real time? Using a unique research design that has involved crowdsourcing popular news articles from both mainstream and suspect news sources that have appeared in the past 24 hours to both ordinary citizens and professional fact checkers, Professor Tucker will report on the individual level characteristics of those likely to incorrectly identify false news stories as true, the results of interventions to attempt to reduce the prevalence of this behavior, and the prospects for crowdsourcing to serve as a viable means for identifying false news stories in real time.
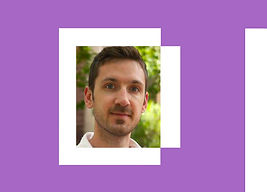
Lee McGuigan
Cornell Tech | Digital Life Initiative
Design Choice: Mechanism Design’s Digital Drift
Mechanism design is a form of optimization developed in economic theory. It casts economists as institutional engineers, choosing an outcome and then arranging a set of market rules and conditions to achieve it. In this paper, Lee McGuigan, Jake Goldenfein, and Salome Viljoen argue that mechanism design, applied in algorithmic environments, has become a tool for producing information domination, distributing social costs in ways that benefit designers, and controlling and coordinating participants in multi-sided platforms.
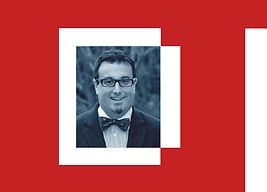
Serge Egelman
International Computer Science Institute | University of California, Berkeley
Taking Responsibility for Someone Else's Code: Studying the Privacy Behaviors of Mobile Apps at Scale
Modern software development has embraced the concept of "code reuse," which is the practice of relying on third-party code to avoid "reinventing the wheel" (and rightly so). While this practice saves developers time and effort, it also creates liabilities: the resulting app may behave in ways that the app developer does not anticipate. This can cause very serious issues for privacy compliance: while an app developer did not write all of the code in their app, they are nonetheless responsible for it. In this talk, I will present research that my group has conducted to automatically examine the privacy behaviors of mobile apps vis-à-vis their compliance with privacy regulations.
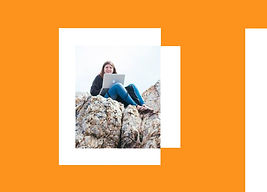
Emma Pierson
Microsoft Research | Jacobs Institute/Cornell Tech (2021)
Modeling COVID with mobility data to understand inequality and guide reopening
In this paper, we develop a model of COVID spread that uses dynamic mobility networks, derived from US cell phone data, to capture the hourly movements of millions of people from local neighborhoods (census block groups, or CBGs) to points of interest (POIs) such as restaurants, grocery stores, or religious establishments.

Cory Doctorow
Author, Activist, Journalist and Blogger
Oligarchy and Technology
Software has eaten the world and crapped out a dystopia: a place where Abbot Labs uses copyright claims to stop people with diabetes from taking control over their insulin dispensing and where BMW is providing seat-heaters as an-over-the-air upgrade that you have to pay for by the month. Companies have tried this stuff since the year dot, but Thomas Edison couldn't send a patent enforcer to your house to make sure you honored the license agreement on your cylinder by only playing it on an Edison phonograph. Today, digital systems offer perfect enforcement for the pettiest, greediest grifts imaginable.

Joseph Turow
Annenberg School for Communication | University of Pennsylvania
Seductive Surveillance and Social Change: The Rise of the Voice Intelligence Industry
Drawing from my forthcoming book The Voice Catchers (Yale U Press, early 2021), I pose two key questions about this new development in the United States: How has the voice intelligence industry been able to gain the kind of social traction that has tens of millions of people giving their up voiceprints to so-called “intelligent assistants”? And in the face of this widespread shift to voice bio-profiling, what social policies should concerned citizens advocate to slow the process and implement regulations regarding this new form of surveillance?
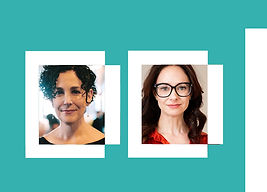
Yaël Eisenstat & Carrie Goldberg
Digital Life Initiative | C.A. Goldberg, PLLC
"With Great Power Comes... No Responsibility?"
Who bears responsibility for the real-world consequences of technology? This question has been unduly complicated for decades by the 1996 legislation that provides immunity from liability to platforms that host third-party content: Section 230 of the Communications Decency Act.

Omid Poursaeed
Cornell Tech
Deepfakes and Adversarial Examples: Fooling Humans and Machines
In this talk, Omid Pouraseed will discuss recent methods for adversarial data manipulation, and mention possible defense strategies against them. Although manipulations of visual and auditory media are as old as media themselves, the recent advent of deepfakes has marked a turning point in the creation of fake content.
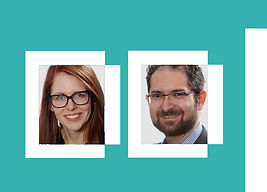
Madelyn R. Sanfilippo & Yan Shvartzshnaider
Princeton University | New York University
Privacy/Disaster: When Information Flows Are Taken Out of Context
Privacy is contextual. Everyday, we manage different contexts and adjust our privacy expectations accordingly. The theory of Contextual Integrity offers a way to capture contextual norms and a heuristic to analyze privacy. This analysis is especially helpful to detect situations in which the system designers take advantage of well-established, contextual privacy expectations, to encourage user disclosures without adhering to governing norms. For example, imagine an app that is marked to you as a patient/doctor communication tool in a medical context, yet it is actually an insurance company trying to get more information on you.

Zachary Chase Lipton
Carnegie Mellon University
Fairness & Interpretability in Machine Learning and the Dangers of Solutionism
Supervised learning algorithms are increasingly operationalized in real-world decision-making systems. Unfortunately, the nature and desiderata of real-world tasks rarely fit neatly into the supervised learning contract.

Michael Sobolev
Cornell Tech
Behavioral Science in the Digital Economy
Over the last decade, behavioral science made significant progress and impact in academic research as well as impacted policy in commercial organizations and governments. At the same time, the rise of digital technologies and the digital economy provides exciting opportunities and presents challenges for the next decade of behavioral science. In this talk, Sobolev will explore novel avenues for behavioral science research in the digital economy.