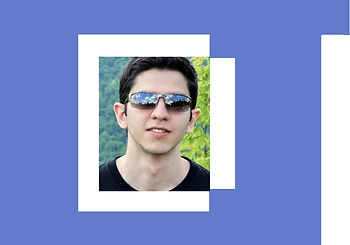
Omid Poursaeed
Cornell Tech
Deepfakes and Adversarial Examples: Fooling Humans and Machines
Abstract
Machine learning models have achieved unprecedented success in numerous challenging tasks. The power of these models can be used both for beneficial and adversarial purposes. Adversarial manipulation of visual content has now become ubiquitous and one of the most critical topics in our digital society. In this talk, I will discuss recent methods for adversarial data manipulation, and mention possible defense strategies against them. Although manipulations of visual and auditory media are as old as media themselves, the recent advent of deepfakes has marked a turning point in the creation of fake content. Powered by the latest technological advances in artificial intelligence and machine learning, deepfakes offer automated procedures to create fake content that is harder and harder for human observers to detect. Visual content can also be manipulated for the purpose of misleading machine learning models. The resulting adversarial examples can significantly degrade performance of the models. Building robust defenses against these attacks is evasive as strong defenses are often beaten by stronger attacks.
About
Omid is a PhD candidate at Cornell Tech working with Professor Serge Belongie. His research interests include Computer Vision, Machine Learning and Generative Models. He studies vulnerabilities of machine learning models in order to make them more robust and less dependent on (private) user data. As a Doctoral Fellow at DLI, he will explore learning models that are both accurate and resilient to various types of adversarial attacks. Omid is also a recipient of Jacobs Fellowship from Cornell University.