DL Seminar | Machines Judging Humans
- Jessie G Taft
- Apr 14, 2020
- 6 min read
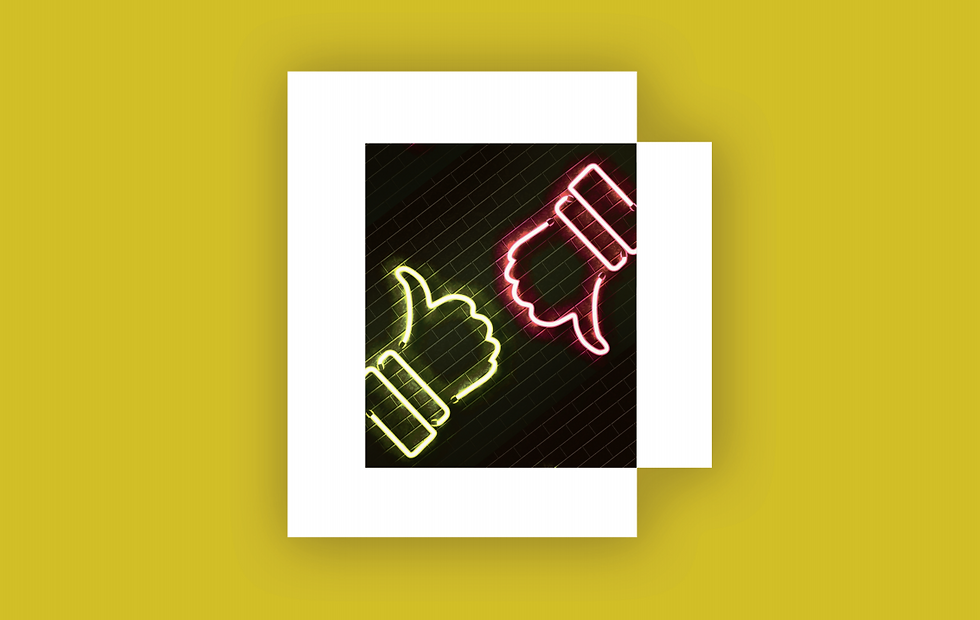
By Aurora Zhang and Julia Hollreiser
On March 26, 2020, the Digital Life Seminar series at Cornell Tech welcomed Professor Frank Pasquale, an expert on the law of artificial intelligence, algorithms, and machine learning at the University of Maryland, to discuss “Machines Judging Humans: The Promise and Perils of Formalizing Evaluative Criteria.” Pasquale’s lecture explored the heated discourse around the waves of algorithmic accountability and the relationship between power and reason in the process of computers judging humans. Beginning with intellectual themes of judgment and calculation and working towards concrete dilemmas that arise in the wake of rapid technological advancements, Pasquale raised an overarching question that remains unanswered: how can we fill the gap between achieving prediction through big data, machine learning, and AI without losing sight of dignity, fairness, and due process?
What’s More Reliable: Judgment or Calculation? Pasquale began the lecture by bringing up locus classic ideas from Joseph Weizenbaum and Blasé Pascal to lay out the theoretic background of algorithmic decision-making. Borrowing Weizenbaum’s juxtaposition of human judgment and computer calculation, Pasquale posits that while any judgment based on individual reasoning admits its innate “weakness” of being an exercise of truth, calculation is expected to produce the “right answer” with big data processing. However, such failure to admit that things “could have been different” is the big problem we now are facing regarding formalized algorithmic evaluation. Although calculation can be conceived as more transparent and more capable of handling issues with massive variables, Pasquale reiterates that there are always problems for which we hope to rely on intuitive human reasoning, rather than mathematical processing.
The “Two Waves” of Algorithmic Accountability
With rising awareness that algorithms can produce discriminatory or biased results, a consensus has developed among academics and policymakers that tech giants should be held accountable for their algorithms. Pasquale refers such positive efforts as the “first wave” of algorithmic accountability, which focuses on reducing unfairness, bias, and discrimination in algorithms. While such efforts definitely should be carried on, the “second wave” of algorithmic accountability is now coming to the stage. Instead of “improving” the existing algorithms, social scientists have begun to ask, “should we use algorithms at all, or as widely?” Despite the obvious tension between the first and second waves, Pasquale noted that they are also complementary, revealing a strong faith in a more emancipatory human-computer interaction system, and algorithms utilization more embedded in the socio-economic context. Envisioning the future, Pasquale implores his audience to reconceive “narrow AI” as a form of intelligence augmentation (IA), an instrument enhancing a human decision-maker, yet never should be the decision maker by itself.
Do Formal Rules Lead to Fairness?
In assessing the quality of any decision, fairness is one of the root indicators. There is a tension in opinions, however, regarding what generates fairness. Pasquale shed light on the relationship of formal rules and fairness from the legal perspective. The legal process conceptions elaborate on how “fairness” requires a host of procedures and institutional check-and-balance, apart from a set of rules. “That is why we turn to simpler algorithms, complex data processing, and scoring,” Pasquale summarized. “Procedural fairness is too expensive, in terms of economy and power.”
Pasquale then zoomed in on scoring and rating systems to illustrate the promises and perils of algorithmic evaluation. Expected to promote informed decision-making, scoring is now under heavy condemnation for its biased results and consequential impact of widening the gap of economic inequality. By looking at different approaches, activists are taking to reform the scoring system in the healthcare and finance industries, and Pasquale again explores the tension and complementarity of the two waves of algorithmic accountability.
The conversation of “scoring” is most advanced in healthcare. As to the inaccuracy and bias exposed, the first-wave proponents strive for collecting more data from under-covered geographical and demographic groups so that economically deprived hospitals can be better represented. In contrast, the second-wave researchers would implore policymakers to be more prudent in introducing scoring systems into a region without considering how it might accelerate the already-existing shortage of healthcare resources.
Regarding algorithms used by the finance industry, Pasquale elaborated on “three troubling versions of financial inclusion”: predatory inclusion, creepy inclusion, and subordinating inclusion. Loans are coded as a “success” by proprietary algorithms as long as they are fully paid back in the end—no matter how the lender suffered in between. When an individual’s financial situation is reduced to a simple binary classification, an algorithm shows its predatory nature in blurring what’s hidden behind the evaluation. For credit scoring, some businesses offer convenient access to credit in exchange for permissive data collection from phones. Such is how “creepy” the financial inclusion can be. The question it raises here is not only that of privacy, but also the nature of data, and if it’s appropriate for evaluating a person’s creditworthiness. A ready example is how companies are found less likely to give a loan to lenders whose social media has content related to political activity. Pasquale reminds us to realize that the underlying ideology is forcing individuals to subordinate their own political rights to avoid economic disadvantage.
Indeed, Pasquale noted, credit scoring provides a good example of first and second wave accountability, as illustrated by the perspectives of rival economists. On one hand, economists like Lawrence Summers are generally enthusiastic about the potential for technology to expand access to credit; many forms of data can provide people with an opportunity to improve their economic stance. On the other hand, however, economists like Darrick Hamilton are more skeptical. While technology provides opportunity, it also generates judgment that mirrors the kind of legal judgment that is often exercised by the State. Hamilton posits that such judgment ought to be transparent and accountable and have forms of due process attached.
A final example of technology that brings both “promises and perils” that Pasquale presented is facial recognition. Facial recognition is starting to become ubiquitous within society and is seen by many as an easy way to identify individuals who do not have a form of identification or to confirm one’s identification. This technology, at first blush, is promising in many contexts, such as in the airline industry where security is paramount. Ultimately, however, facial recognition carries the worry that it can fully eviscerate people’s privacy. Pasquale thus dubbed facial recognition the “plutonium of AI,” as it has the power to do great good while also threatening extensive social costs.
Embedding Technological Innovation in Larger Societal Narratives
Ultimately, Pasquale proposed, economic analysis boils down to narratives about how society is supposed to work. As an illustration, Pasquale focused his discussion on the emergence of platform capitalism and his theory that platform capitalism presents two narratives: the conventional narrative and the counter-narrative. The conventional narrative surrounding platform capitalism is that platforms such as Uber and Task Rabbit present societal promises. For instance, they promote fairer labor markets by enabling lower-cost entry into their respective markets, and they reduce the impact of discrimination by increasing the number of service providers in their markets. The counter-narrative, however, highlights their perils. These platforms arguably entrench existing inequalities in the market and promote precarity by reducing workers’ bargaining power and employment stability, and they increase discrimination by identifying customers with picture-based profiles.
Rival narratives go beyond platform capitalism, however. Consider credit scoring: is its narrative one of borrower redemption, or one of borrower subordination? Now consider facial recognition: is its narrative one of protection, or one of abusive over-surveillance? Pasquale does not promote either the conventional narrative or the counter-narrative as being the “right” lens in any instance. Rather, he suggests that developing both of these narratives can help set up social scientific inquiry and promote rhetorical engagement.
Algorithmic accountability has been a rising concern throughout the last decade as social scientists, data scientists, lawyers, and journalists have exposed tensions between technology and our fundamental concepts of dignity, fairness, and due process. Pasquale implores us to remember that while the “first wave” of algorithmic accountability work has targeted existing issues, we must not forget about broader structural concerns that can only be tackled through a “second wave” of algorithmic accountability. Technology is often at odds with our classic concepts of fairness, and it is our duty to create a more desirable fit between technology and our societal systems.
Aurora Zhang is a MS student at Cornell Tech. Julia Hollreiser is a JD candidate at Cornell Law School.
Regards for the purpose of post this amazing piece of writing! I recently came across yuor web blog perfect for your preferences. It includes marvelous not to mention advantageous items. Cultivate monetary management give good results! code promo gratuit 1xbet
Thank you for helping people get the information they need. Great stuff as usual. Keep up the great work!!! https://grandgoldman.com/
Outstanding article! I want people to know just how good this information is in your article. Your views are much like my own concerning this subject. I will visit daily your blog because I know. It may be very beneficial for me.Alexistogel
this is really nice to read..informative post is very good to read..thanks a lot! olxtoto this is really nice to read..informative post is very good to read..thanks a lot! edctoto this is really nice to read..informative post is very good to read..thanks a lot! rogtoto login this is really nice to read..informative post is very good to read..thanks a lot! olxtoto this is really nice to read..informative post is very good to read..thanks a lot! olxtoto alexistogel
Great Information sharing .. I am very happy to read this article .. thanks for giving us go through info.Fantastic nice. I appreciate this post.togel online terpercaya