DL Seminar | Data Access & AI Explainability
- Digital Life Initiative
- Apr 11, 2024
- 9 min read
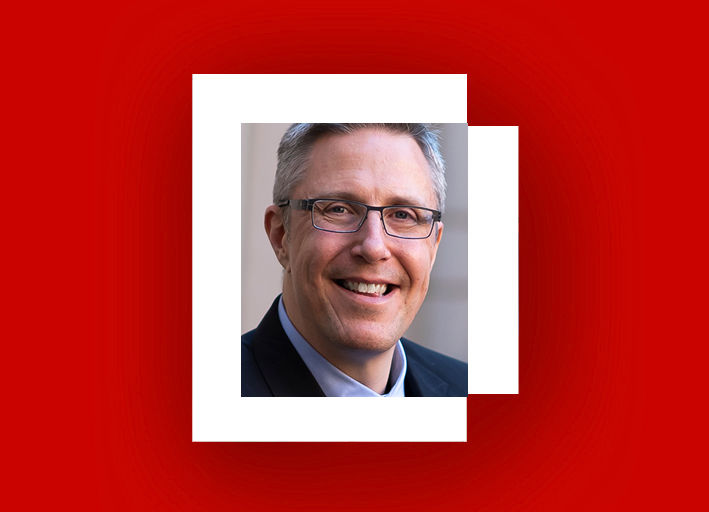
Individual reflections by Daniel Dolnik, Tian Jin and Yiran Li (scroll below).
By Daniel Dolnik
Cornell Tech
At our decent DLI Seminar we had the pleasure of hearing from Cornell’s own Professor Frank Pasquale who teaches on the Law of AI. The seminar was an introduction to Professor Pasquale’s goal of bringing to a wide academic audience the large problem of data access and AI explainability, which continues to get more relevant with each passing day.
Professor Pasquale’s talk began with a woman Mary Ebeling who suffered a miscarriage and then for years proceeded to have a continued digital presence as advertisers and others proceeded to send her mailings for her supposed child. Despite her best efforts, Mary was not able to track down the source of this false information or eliminate it, and wrote a book about her experience. Mary’s experience appears emblematic of the general issues surrounding data access and tracking. As professor Pasquale argued, the problem has only gotten worse while the technology continues to get better and more pervasive.
Keeping track of where all of our data comes from and where it goes to is a contentious topic and as governments look to tackle this issue, there is a split between the privacy of individuals on one hand, and protecting secret corporate algorithms and thus potentially their innovations on the other. Generally, Europe has been falling on the side of individual privacy, while the US, with the notable exception of California, has firmly prioritized corporate secrecy.
Professor Pasquale made it clear that ‘Big Data’ has many positives as well. It can broaden the scope of evaluations and offer more opportunities for people to demonstrate merit rather than be judged by just one or two metrics. However, it can be misused in many ways. It also often contains inaccuracies; for example, if some category of data is 51% predictive, that might be enough for companies to derive value from it on the whole, but harms the 49% of individuals who ended up on the wrong side of the equation. Additionally, there are many inappropriate types of data being used that can lead to various forms of discrimination, even if they are not as clear as sex or race.
When tackling the issue of explainability, even assuming that we agree that individuals should have some rights to see how their data is used, there are still many questions remaining. In a hypothetical scenario where someone is being evaluated in a credit application, and there is a corresponding data access request to see how the decision was made, the company could provide anything from a high level overview down to the inner working of the algorithms themselves, which might be looking at hundreds or thousands of data points for any one person. Additionally, as models get more complex, there may not even be a clear answer as to which piece of information was the deciding factor, and there might be multiple levels of interaction. This is something that regulators should think carefully about and may depend on the specific industry or task.
In current attempts to regulate data use, most of the effort has been spent looking at the costs of regulation rather than the benefits, as those can often be more amorphous and hard to quantify. However, sticking to only what can be quantified (i.e. a cost of some regulation is estimated to be $10M, and therefore is not pursued) leaves out the much less tangible benefits to individuals and to society, and can take advantage of false precision. Professor Pasquale’s goal is a more wholistic evaluation of the benefits of data protection and information access rights. These benefits could then be evaluated in conjunction with costs of regulation in either an informal cost-benefit analysis, or a scenario analysis of what sort of big picture scenarios different regulations might lead to.
Ultimately, while not intending to offer a definitive answer to these complex questions, Professor Pasquale suggested that governments need to:
1) Track how the data-informed evaluation of persons is progressing.
2) Create regulation to clarify individual rights.
3) Look for ways to move beyond false formality in the analysis of regulatory costs and benefits.
The participants in the DLI lecture brought up many interesting points in response to the lecture. One question concerned the formalization of a qualitative cost-benefit analysis, which Professor Pasquale said would require a dedicated community and practice of evaluators that build up intuitions over time.
Another question cited the idea of potential costs to extreme algorithmic transparency, where someone might be able to game the system if they knew exactly how it worked. Lastly, there was a question of how we would know if ‘explainability’ for a given algorithm was actually accurate. In Professor Pasquale’s argument, if the system is so complex or fragile that it can’t be easily explained, that raises the question of whether we should be using it at all to make socially relevant decisions.
This was a fascinating conversation that is sure to stay on the forefront as AI continues to become more mainstream and systems continue to get more complex and data-heavy. Personally, I can see a very real trade off here, as Europe seems to be falling behind the USA in terms of AI development; and ultimately whoever is leading the technology race might be the one whose input matters most. Regulations need to be carefully constructed to protect individuals, while also being reasonable about the costs imposed on innovation as a result, and likely need to vary across industries.
Having come from the world of finance prior to my time at Cornell Tech, I have seen the value of both human-understandable models, and the value that more data-driven approaches can bring, and this is a balance that I have personally seem in conflict for many years in my work setting. I have also seen some clear win/win scenarios, like effective algorithms in specific domains that can still be well-understood by humans, and in my opinion those are the holy grail. Unfortunately those algorithms are hard to come by and it is often easier to just throw all of the data into a large soup when creating a model, without much consideration for how it is used and whether anyone can understand it. This is something that AI developers themselves should be thinking about.
Ultimately Professor Pasquale’s work here is very important, and regardless of what regulations get implemented, it is important both for policy-makers and for us as individuals to be thinking deeply about these questions. It will be critical to have a well-structured framework and think about potential trade-offs around data use with clarity, as these issues are sure to play a very big role in what our society looks like going forward.
By Tian Jin
Cornell Tech
The talk provided a thorough exploration of the implications of algorithmic scoring and data privacy in our rapidly evolving digital society. Beginning with the elaboration of algorithmic lenders differing from traditional ones, prof. Pasquale introduced a frontier creditworthiness assessment based on expansive data. Despite the comprehensive understanding of individuals via data points, the dual nature of big data naturally brings in perils of potential inaccuracies, inappropriate considerations and new forms of discrimination. Therefore, robust regulatory frameworks on data access and AI explainability are in urgent need to navigate those issues.
Speaking of the regulation, prof. Pasquale highlighted ethical concerns, especially around AI-driven assessments of consumer financial reputations. This includes accuracy, representativeness, and the impact of data discrimination. By introducing regulations like California Consumer Privacy Act (CCPA) and California Privacy Rights Act (CPRA) in California, the talk illustrated some historical attempts to regulate these areas, but also the limitations of those attempts, suggesting that new models are needed to incorporate public values into technological advancements. In a practical example of algorithmic scoring, the talk demonstrated how a hypothetical individual’s financial risk profile might
be constructed by AI, which also illustrates some inherent challenges like data cleaning and the implications of outlier exclusion.
In order to evaluate regulations, the talk then introduced SRIA, which is an initial economic
evaluation of regulatory changes to businesses and individuals. Despite the function of quantifying the costs and benefits, prof. Pasquale also mentioned the criticism of its tendency to overemphasize quantifiable outcomes and undervalues non-monetary benefits like fairness and social equity. Following such discussion, the talk ends up with an emphasis on the advancement of data-informed evaluations and the necessity of rethinking policy evaluation. Both formal and informal analyses and comprehensive recognition of the full range of benefits and costs associated with data rights and privacy regulations are encouraged and worth further reflection.
Reflecting on the content of the talk, the larger digital life landscape has become increasingly
influential in our daily life. Our personal data has gradually become a currency of its own, whose collection and application has already gone far beyond our attention. The distinction between online and offline identity blurs, with digital footprints informing decisions that have real-world impacts on access to credit, employment, and so on. Just as the example of algorithmic scoring indicates in the talk, as AI and machine learning continue to advance, related ethical considerations become severely crucial and urgently require a balance between leveraging data for innovation and safeguarding individual privacy and fairness.
Moreover, I was impressed by the talk’s emphasis on regulatory evaluation and responses to the management of big data, especially when data breaches and misuse of information are common in the current digital era. On one hand, regulatory agencies, on behalf of public interest and privacy rights, should look beyond the monetary costs of regulations while looking for broader socioeconomic benefits and individual rights. On the other hand, users’ rights to privacy are often at odds with the commercial objectives of businesses, therefore such regulation is of great significance. Thriving on the free flow of information, regulatory actions indicate our struggles to assert control and ownership over personal data in the modern digital ecosystem.
As digital technology continues to permeate every aspect of our lives, this talk serves as a compelling argument for the careful consideration of how we evaluate and manage the use of personal data, ensuring that progress in the digital age does not come at the expense of fundamental human rights.
By Yiran Li
Cornell Tech
The presentation I attended focused on the significant implications of data access and explainability, underscoring their vital roles in sculpting our digital environment. It methodically outlined key considerations that are essential for maintaining ethical standards and regulatory compliance in data management.
Firstly, the discussion shed light on the myriad harms associated with restricted access to personal data, particularly emphasizing the severe repercussions of data privacy violations. By delving into the complexities of how personal data is used in algorithmic lending, the presentation highlighted a critical example set by Bruckner. It revealed how lenders utilize a broad spectrum of personal information—including email addresses, social media connections, and even text messaging patterns—to assess creditworthiness. This practice raises significant ethical questions, illustrating the potential for misuse of personal information and underscoring the necessity for strict regulatory frameworks to prevent discriminatory practices and protect individual privacy rights.
In this vein, the example of California's data access rights was discussed as a pioneering model within the United States. California’s legislative approach, particularly through the California Consumer Privacy Act (CCPA), exemplifies robust data privacy regulation. The presentation argued for the adoption of similar measures at the national level to ensure a uniform standard of privacy protection that could simultaneously foster innovation and economic growth without compromising individual privacy.
Moreover, the presentation explored the ramifications of opaque algorithmic decision-making processes through a Consumer Scoring Case Study. It posited a scenario where Bob, a hypothetical consumer, is denied credit without clear justification. The case study demonstrated how common practices in data management, such as data cleaning, could inadvertently misrepresent an individual's digital persona, leading to biased decision-making outcomes. The use of questionable data sources, like arrest records in credit scoring, was criticized for perpetuating discrimination and widening existing social inequalities.
The discussion also introduced the concept of Modeling Algorithmic Evaluations of Persons, emphasizing the critical need for transparency in the algorithms that increasingly influence many aspects of our lives. It was noted that different levels of disclosure, from model-centric to subject-centric, could greatly enhance the transparency of these processes. At higher levels of disclosure, consumers like Bob would be able to understand and potentially challenge the data points and methodologies influencing algorithmic decisions, thereby ensuring a higher degree of fairness and accountability.
Lastly, the presentation proposed a framework for policy evaluation aimed at determining the appropriate level and scope of information access in data governance. This framework is intended to guide informed decision-making and encourage the implementation of practices that ensure transparency and accountability. Highlighted within this framework was the use of standardized regulatory impact assessment, a tool that systematically assesses the benefits and costs of regulatory decisions. However, it was critiqued for its overemphasis on cost considerations at the expense of a comprehensive evaluation of benefits, suggesting a need for a more balanced approach in evaluating the overall impact of data governance policies. This approach often overlooks the potential benefits, and since the benefit is not always easily quantifiable, a more nuanced analysis is required to capture the full spectrum of positive outcomes that effective data governance can bring.
In summary, the presentation emphasized the critical importance of data access and explainability in shaping our digital landscape, showcasing the ethical concerns and regulatory frameworks such as California's CCPA to safeguard privacy rights. Additionally, it proposed a comprehensive policy evaluation framework, acknowledging the necessity for balanced assessment.