DL Seminar | AI, Fair Use, and Power
- Jessie G Taft
- Feb 13, 2018
- 3 min read
Updated: Jan 8, 2019
By Margot Hanley | Sociology Student (Columbia University)

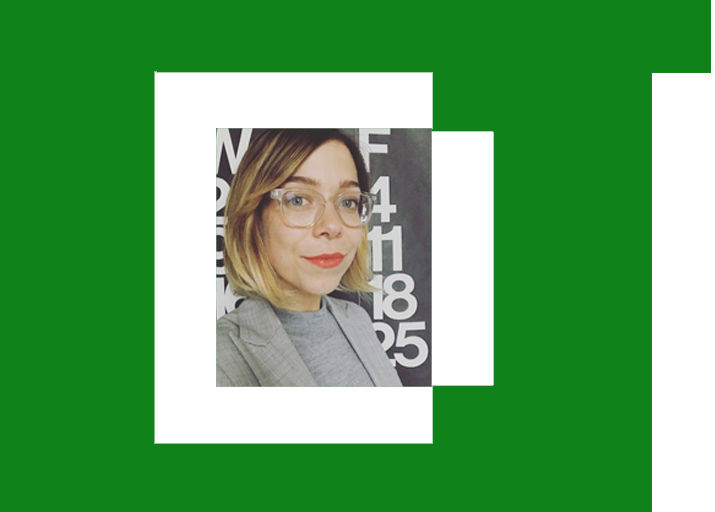
One of the lesser known causes of bias in AI training data, and potentially one of the most significant ones according to NYU’s Amanda Levendowski, is copyright law. Copyright, she argues, plays a huge role in creating an unequal allocation of the training sets that make AI valuable. This reality perpetuates inherent bias, while also entrenching the competitive advantage of incumbent tech giants. In an optimistic and ironic twist, however, she suggests that copyright law may also offer a solution, in the form of the doctrine of “fair use.”
Giving a crash course on the history and practice of copyright law in AI, Levendowski framed changes in modern licensing as a matter of practicality: whereas an anthologist compiling a book might pay ten poets a small fee each to license their poems, a large tech company might have to pay for the millions of photographs necessary to make image-recognition AI systems successful. While the volume of “works” has increased enormously, the philosophy remains the same; a photo used to train AI, just like a poem in an anthology, is a product of human expression, and should – one presumes – be considered as such.
The impact of copyright law becomes especially important when considering the consolidation of market power in the hands of seven companies: Apple, Baidu, Google, DeepMind (owned by Google), Facebook, IBM, and Microsoft. These digital goliaths generally source the massive amount of training data required to develop AI systems in one of two ways: via the “build-it model” or the “buy-it model.” They can either build or create copyright material from their users’ submissions (like Facebook’s access to the copyrighted works of its two billion users), or leverage their enormous reserves to buy copyright data from someone else (like IBM).
Buying or building is often infeasible for small companies, and they may consequently turn to less reputable options. Such data is easily available and perceived (accurately or not) as being low-risk: even if this data is copyright protected, there’s a slim chance that someone would send a cease-and-desist order or take-down notice for copyright infringement. Companies that engage in such dubious data collection practices can even cover their tracks by being acquired by a large incumbent company – in turn offering a regulatory loophole to their eventual owners. The small companies that want to avoid committing copyright infringement or dubious data collection may instead opt to train their AI on data available in the public domain, like the Enron emails. This data – while convenient and legal – is often not the cleanest, most current, or most rigorously-collected data available and, as such, tends to be rife with biases. Ultimately, when copyright law obstructs data accessibility, Levendowski’s argument is that it ensures skewed, unrepresentative AI algorithms and technologies.
For Levendowski, there may be a solution to these problems via the doctrine of fair use, which identifies contexts when it is legal to use copyright material without the copyright holder’s permission. A classic example of fair use is a teacher presenting copyright works in a classroom. Levendowski argues that if fair use enabled broader access to copyright data, it could be instrumental in supporting competition, innovation, accessibility, and fairness.
This claim about applying fair use to a new technological use-case, though intriguing, generates a whole new set of questions. Is it realistic to imagine that legalizing the appropriation of copyright material would have made more and fairer recommendation engines? Are there really companies out there with the heft and drive to take on the tech giants? Does relaxing copyright law help new players more than it helps incumbents? Does invoking fair use to protect the broader public interest risk diluting the personal rights of creators? And what about more granular data – not creative works, as such, but just the digital trails of our daily activities? At the broadest level, how do we balance the interests of the public and the individual in the case of copyright law?
There is no silver bullet for the litany of issues arising in AI, and fair use is no exception. It is only through bringing proposed solutions to the table, as Levendowski has, that we can meaningfully assess their promise and determine whether they can genuinely deliver competitive, accessible, and ethical technological advances in the public interest. Within this calculus, it is important that we not be naïve about the entrenched power of the digital goliaths, nor forget that there won’t be very much for machine learning to learn from if we don’t continue to respect and nourish creators. The profound challenge that AI presents to copyright is whether it’s possible to find a way of both harnessing and honoring the power of human creativity.