Digital Eyes on the Street
- Digital Life Initiative
- Apr 15
- 7 min read
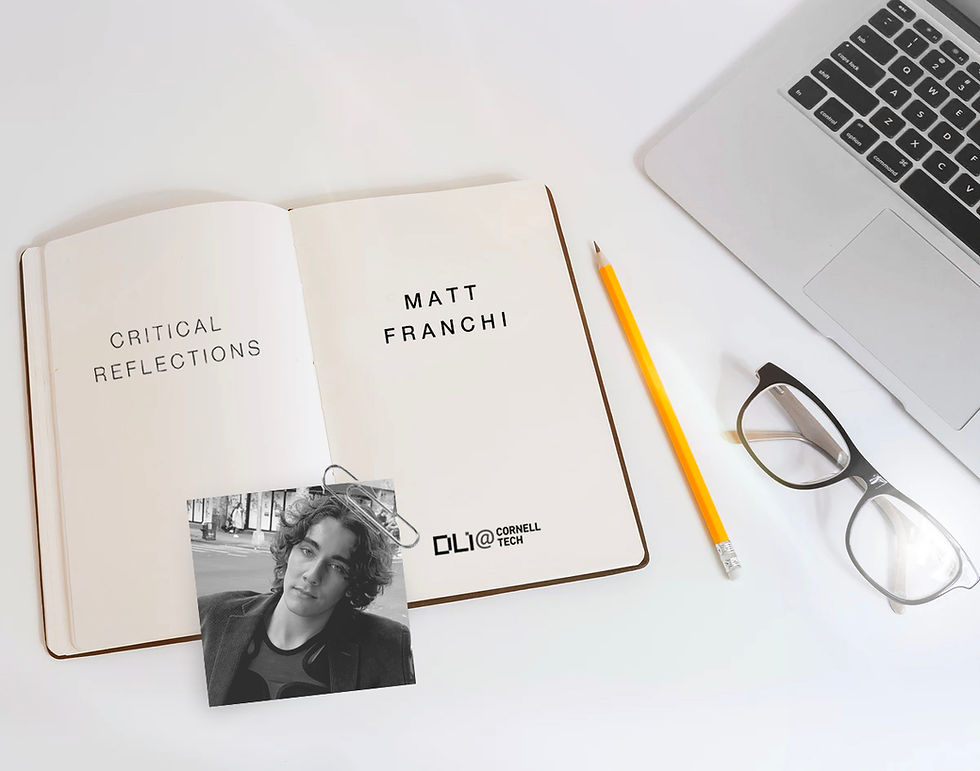
By Matt Franchi (Cornell Tech)
On December 4th, 2024, I opened up CityStream Live [1] and searched for evidence. At 6:44 AM, Brian Thompson, the Chief Executive Officer of UnitedHealthcare, had been shot in Midtown Manhattan. The incident set off an enormous search for the gunman, now alleged to be Luigi Mangione. On my laptop, I sleuthed through imagery emitted by networked dashcams (mostly attached to ridesharing vehicles) on the nine streets adjacent to the New York Hilton Midtown hotel and found imagery showing signs of a crime (police lights, blockades, and empty streets), but no sightings of the gunman. Several days later, authorities had leveraged NYC’s extensive network of CCTV cameras to trace Mangione’s location at seven points the day of the shooting (News, 2024). The tracing was thorough, but delayed, showing me that while NYC is well-covered by a network of static cameras, that network is fragmented. New, monolithic tools are emerging without this constraint.
On the street, there are sweeping changes in information flows about. The famous urbanist Jane Jacobs proffered the theory of ‘eyes on the street’, where neighborhood vitality and safety are anchored under the watchful eyes of local community members (Jacobs, 1961). Jacobs assumed sentient eyes; her heyday in the 1960s preceded the propagation of the first artificial watchmen, the CCTV camera (Williams, 2003).
In the past 50 years, new technologies with joint capabilities for tracking and monitoring, aggregation and analysis, and dissemination and publication (Nissenbaum, 2009) have yielded an explosion of digital eyes on the street. Today, on a walk in the city, I see CCTV cameras, body-worn cameras on NYPD officers, traffic enforcement cameras, smart doorbells, and smartphones. Even the MTA buses have outward-facing cameras on them for automated mobile bus lane enforcement. If I look more closely, I see stickers on the passenger windows of ridesharing vehicles, warning would-be passengers that the driver uses an internet-connected dashboard camera (dashcam). As seen anecdotally, digital eyes come in many variants; here, I’ll focus on an important factor in that variance: mobility.
First, static, immobile cameras and sensors become fixtures of the built environment; unless intentionally hidden, their presence is registered and accounted for in the minds of community members. Take the 32-foot tall 5G cellular towers being installed around NYC, which have sparked outcry from residents, conspiracists, and preservationists, despite the implications for better network connectivity (Stewart, 2024); however, 5G towers are derided mostly for their aesthetic and size, instead of their ability to surveil users. Similarly with CCTV, research has shown that some societies have adjusted to a reality of surveillance, especially in public contexts like public transport and shopping malls (van Heek et al., 2017). Overall, static sensors and cameras have been studied extensively in the literature (Slobogin, 2002), and so I will focus more so on the emergent paradigm of mobile sensors.
While fixed sensors become permanent fixtures in the urban landscape, mobile cameras and sensors introduce a fundamentally different digital surveillance paradigm—one characterized by temporary, transient observation. Today’s mobile sensors, including dashcams and body-worn cameras, are usually connected to a central network. Then, for owners of the network and others with access, visual streams from all connected sensors are visible in near-realtime. With increased scale, this is a shift that matters significantly for privacy, especially as other hardware capabilities are introduced.
For example, I work with a specific paradigm of mobile sensors (networked dashcams) that are well-deployed in American cities. These dashcams are attachable, lightweight, networked, instructible, and optionally intelligent cameras that transmit photographic or inferential streams of data from the perspective of a mobile bearer to an aggregated, digital source. Images produced by networked dashcams are tagged with accurate timestamps and geographic coordinates. The bearer’s consent to new informational flows posed by dashcams is rooted in the act of attachment/detachment. At-scale, these sensors allow remote visual inspection and the mining of trends, from individual all the way to city-scale level. This mining of trends at many granularities is only permitted by the dense street imagery (DSI) that a sufficiently-large network of these sensors can produce.
A key worry that may ignite with increased, and more varied, usage of DSI is the upturn in temporal density; increasingly more locations without streams of continuous surveillance from static sensors will go from being depicted a few times a year to several times per day, or in urban environments, several times per hour. In 2019, the MIT Senseable Cities lab analyzed empirical taxi trip data, finding that only 1,179 trips would be necessary to ‘scan’ half of NYC’s street segments (O’Keeffe et al., 2019). Networked dashcams are now installed on thousands of ridesharing vehicles throughout the city. Further, there are ethical dimensions beyond privacy. New advancements may bring about new discourse around consent, perpetuate algorithmic biases in image processing, or have unintended effects on public behavior.
Mobile sensors lie in between CCTV and robotic technologies like autonomous vehicles. They assume the movement profile of whatever they are attached to, rather than having an independent ability to move about and interact with the environment – a relationship of commensalism. In the case of vehicle dashcams, only views along driver-chosen routes can be depicted. In the case of smart glasses, perspective is literally skimmed off the wearer.
Economies-of-scale and remaining technical overhead in robotics mean that mobile sensors are the concurrent driver behind the emerging norm of ‘digital eyes on the street’, instead of downstream technologies like autonomous vehicles, drones, and sidewalk robots. Further, despite being passive sensors that can only depict their bearer’s chosen surroundings, with enough connected sensors (O’Keeffe et al., 2019), this passively-powered apparatus morphs into something akin to a ‘sidewalk observatory’, allowing for active and targeted curation, tracking, and analysis.
This shift from human to digital observation represents a fundamental transformation of Jacobs' original 'eyes on the street' concept. Context-relative informational norms from ‘digital eyes on the street’ inherit, of course, from Jacobs’ earlier ‘eyes on the street’ theory, surveying methods from urban planning & sociology, and human-automobile interactions. I won’t pursue a full tracing of these norms here, but it is important to note that there is a prima-facie violation of the above norms when the perception is recorded (Nissenbaum, 2009), often permanently; data providers, perhaps implicitly cognizant of this, are investigating a shift to on-edge processing (Shi et al., 2016), where inferences about incoming footage are made and transmitted directly on the device, and raw visual footage is discarded. This nascent trend further complicates the landscape, and future work remains to disentangle the appropriateness of the information flows that constitute ‘digital eyes on the street’.
The evolving landscape of mobile surveillance technologies raises important questions about appropriate research methodologies and ethical applications. Considerate uses of the ‘sidewalk observatory’ produced by DSI are bias-aware and privacy-aware. In my work, I apply inferential techniques (including artificial intelligence and machine learning based methods) to dense street imagery from networked dashcams. My work is bias-aware in that inferences are not taken at face value; we develop post-processing methods (like spatial Bayesian models) that give principled measures of uncertainty, and attempt to reconcile with external covariates, when they exist. My work is privacy-aware in that I’ve selected activistic applications (e.g., turning cameras back on the police) and applications that focus on physical infrastructure, and have pursued audits of dense street imagery that call attention to existing weaknesses, including the easy inference of group membership even under pedestrian obfuscation.
I believe that this research is appropriate, due to myself, an academic researcher, being the receiver; Nexar, a startup who I have a longstanding collaborative relationship with, being the sender; dense street imagery, from Nexar dashcams, being the information transferred; a transmission principle involving confidentiality, temporality, exchange, and notice; and, finally, subjects that either fall under the built environment, entities who themselves conduct surveillance, or pedestrian-focused analyses that aggregate and avoid making stratifying classifications. However, it remains to be seen if society would agree; individual components of the above tuple are being assessed by current research (e.g., societal norms around wearing smart glasses in public (Kaviani et al., 2024)).
Finally, to forecast, there are emerging technologies that will make information flows involving mobile sensors absolutely ubiquitous (Due, 2014), most notably in urban environments (Jensen, 2016). Already, the Pareto frontier representing the trade-off between spatial and temporal coverage is expanding rapidly (O’Keeffe et al., 2019), with new technologies enabling both broader area coverage and more frequent observations, simultaneously. Smart glasses, sidewalk robots, and drones will produce more and more dense street imagery. I’m sure more sources will come about, as well.
All said, there is friction against a future resemblant of Yevgeny Zamyatin’s We (Amey, 2005), including corporate interests against the sharing of data and legislative policy that restricts data collection. Academics, journalists, and the public sector can provide more friction. As the technologies that enable the generation of DSI grow, it is paramount to consider privacy, and, more fundamentally, governance, pre-emptively, rather than post-hoc as with technologies like Google Street View. GSV permits identifiable depiction at a singular place, in a singular moment in time. Untethered, DSI might soon permit identifiable depiction at any place, at any moment in time.
[1] CityStream Live is an online platform where users of Nexar Inc.’s data products can view recently-sampled images from networked dashcams, on an interactive map.
References
Amey, M. D. (2005). Living Under the Bell Jar: Surveillance and Resistance in Yevgeny Zamyatin’s “We.” Critical Survey, 17(1), 22–39.
Due, B. L. (2014). The future of smart glasses: An essay about challenges and possibilities with smart glasses (Vol. 1). Centre of Interaction Research and Communication Design, University of …. https://circd.ku.dk/circd-publishing/An_essay_about_the_future_of_smart_glasses.pdf
Jacobs, J. (1961). The Death and Life of Great American Cities. Vintage Books.
Jensen, O. B. (2016). New “Foucaultdian Boomerangs”: Drones and Urban Surveillance. Surveillance and Society, 14(1), 20–33.
Kaviani, F., Lyall, B., & Koppel, S. (2024). Exploring social perceptions of everyday smartglass use in Australia. PLOS ONE, 19(11), e0313001. https://doi.org/10.1371/journal.pone.0313001
News, A. B. C. (2024). UnitedHealthcare CEO shooting suspect’s timeline before, during, after the brazen murder. ABC News. https://abcnews.go.com/US/unitedhealthcare-ceo-shooting-suspects-movements-timeline/story?id=116504579
Nissenbaum, H. (2009). Privacy in Context: Technology, Policy, and the Integrity of Social Life. Stanford University Press. https://doi.org/10.1515/9780804772891
O’Keeffe, K. P., Anjomshoaa, A., Strogatz, S. H., Santi, P., & Ratti, C. (2019). Quantifying the sensing power of vehicle fleets. Proceedings of the National Academy of Sciences, 116(26), 12752–12757. https://doi.org/10.1073/pnas.1821667116
Shi, W., Cao, J., Zhang, Q., Li, Y., & Xu, L. (2016). Edge Computing: Vision and Challenges. IEEE Internet of Things Journal, 3(5), 637–646. IEEE Internet of Things Journal. https://doi.org/10.1109/JIOT.2016.2579198
Slobogin, C. (2002). Public Privacy: Camera Surveillance of Public Places and the Right to Anonymity. Mississippi Law Journal, 72(1), 213–316.
Stewart, D. (2024, June 10). Does New York City Really Need These Giant 5G Towers? The New York Times. https://www.nytimes.com/2024/06/10/nyregion/street-wars-new-york-city-5g-towers.html
van Heek, J., Arning, K., & Ziefle, M. (2017). The Surveillance Society: Which Factors Form Public Acceptance of Surveillance Technologies? In M. Helfert, C. Klein, B. Donnellan, & O. Gusikhin (Eds.), Smart Cities, Green Technologies, and Intelligent Transport Systems (pp. 170–191). Springer International Publishing. https://doi.org/10.1007/978-3-319-63712-9_10
Williams, C. A. (2003). Police Surveillance and the Emergence of CCTV in the 1960s. Crime Prevention and Community Safety, 5, 27–37.
Matt Franchi
DLI Doctoral Fellow
Cornell Tech
Cornell Tech | 2025